Background¶
This notebook provide an alternative filter-and-fire pytorch neuron model that might be easier and more robust to use.
In fact, we provide here an implementation of the Exp 3. that was introduced in Filter-and-Fire_Neuron_Model_SNN.ipynb, but wasn’t implemented there.
Experiments conducted (23.7.23)¶
Note:
M: number of connections per presynaptic axon
Exp 1. M = 1
Exp 2. M = 3 (Exp 3. of Filter-and-Fire_Neuron_Model_SNN.ipynb)
Imports¶
!pip install tqdm
import numpy as np
import matplotlib.pyplot as plt
import matplotlib
import torch
import torch.nn as nn
from scipy import signal
from tqdm.auto import tqdm as pbar
dtype = torch.float
# Check whether a GPU is available
if torch.cuda.is_available():
device = torch.device("cuda")
else:
device = torch.device("cpu")
my_computer_is_slow = True # set this to True if using Colab
Requirement already satisfied: tqdm in /ems/elsc-labs/segev-i/ido.aizenbud/.local/lib/python3.9/site-packages (4.62.3)
Hyperparameters¶
# Constants
SECOND = 1
MS = 1e-3
HZ = 1
PI = np.pi
DT = 1*MS # large time step to make simulations run faster
ANF_PER_EAR = 100 # repeats of each ear with independent noise (was 1000 in my other notebooks)
ENVELOPE_POWER = 2 # higher values make sharper envelopes, easier
RATE_MAX = 600*HZ # maximum Poisson firing rate
F = 20*HZ # stimulus frequency
DURATION = .1*SECOND # stimulus duration
DURATION_STEPS = int(np.round(DURATION/DT)) # 100
T = np.arange(DURATION_STEPS)*DT # array of times
# Network
INPUT_SIZE = 2*ANF_PER_EAR # 200 input neurons
NUM_HIDDEN = 30
NUM_CLASSES = 180//15 # classes at 15 degree increments
print('Number of classes = {}'.format(NUM_CLASSES))
TAU = 5*MS # this used to be 20 in the SNN Starting Notebook, is now 5 in Quick Start Notebook
# Training
MY_COMPUTER_IS_SLOW = True
if MY_COMPUTER_IS_SLOW:
BATCH_SIZE = 64
N_TRAINING_BATCHES = 64
else:
BATCH_SIZE = 128
N_TRAINING_BATCHES = 128
N_TESTING_BATCHES = 32
NUM_SAMPLES = BATCH_SIZE*N_TRAINING_BATCHES
BETA = 5 # for Surrogate Gradient Descent
NUM_EPOCHS = 100 # (takes ~6min/epoch with INPUT_SIZE=200)
LR = 0.0001
# Filter-and-Fire Neuron Model (Beniaguev et al., 2022)
NUM_AXONS = 100
CONNECTIONS_PER_AXON = 3
NUM_SYNAPSES = CONNECTIONS_PER_AXON * NUM_AXONS
Number of classes = 12
Functions¶
Stimulus¶
def input_signal(ipd, usual_phase_delays=True):
"""
Generate an input signal (spike array) from array of true IPDs
Parameters:
ipd (array): true IPDs
Returns:
spikes (array): input signal from true IPDs (spike trains)
"""
num_samples = len(ipd) # i.e., NUM_SAMPLES
phi = 2*PI*(F*T+np.random.rand()) # array of phases corresponding to those times with random offset
# each point in the array will have a different phase based on which ear it is
# and its delay
theta = np.zeros((num_samples, DURATION_STEPS, 2*ANF_PER_EAR))
# for each ear, we have anf_per_ear different phase delays from to pi/2 so
# that the differences between the two ears can cover the full range from -pi/2 to pi/2
if usual_phase_delays: # Exp 1., Exp 2.a
phase_delays = np.linspace(0, PI/2, ANF_PER_EAR)
else: # Exp 2.b, Exp 3.
phase_delays = np.linspace(0, 0, ANF_PER_EAR)
# now we set up these theta to implement that. Some numpy vectorisation logic here which looks a little weird,
# but implements the idea in the text above.
theta[:, :, :ANF_PER_EAR] = phi[np.newaxis, :, np.newaxis]+phase_delays[np.newaxis, np.newaxis, :]
theta[:, :, ANF_PER_EAR:] = phi[np.newaxis, :, np.newaxis]+phase_delays[np.newaxis, np.newaxis, :]+ipd[:, np.newaxis, np.newaxis]
# now generate Poisson spikes at the given firing rate as in the previous notebook
spikes = np.random.rand(num_samples, DURATION_STEPS, 2*ANF_PER_EAR)<RATE_MAX*DT*(0.5*(1+np.sin(theta)))**ENVELOPE_POWER
return spikes
# Generate some true IPDs from U(-pi/2, pi/2) and corresponding spike arrays
def random_ipd_input_signal(num_samples, usual_phase_delays=True, tensor=True):
"""
Generate the training data: true IPDs are in U(-pi/2, pi/2)
Parameters:
num_samples (int)
usual_phase_delays (boolean): flag on the use of usual or null phase delays
tensor (boolean): flag on the use of tensor or numpy objects for the objects returned
Returns:
ipd (array): true IPDs from U(-pi/2, pi/2)
spikes (array): input signal corresponding to the true IPDs in the training data
"""
ipd = np.random.rand(num_samples)*PI-PI/2 # uniformly random in (-pi/2, pi/2)
#print(ipd)# okay
spikes = input_signal(ipd, usual_phase_delays)
#print(spikes) # empty
if tensor:
ipd = torch.tensor(ipd, device=device, dtype=dtype)
spikes = torch.tensor(spikes, device=device, dtype=dtype)
return ipd, spikes
def discretise(ipds):
"""
Discretise the ipds in the training data
Parameters:
ipds (tensor)
Returns:
tensor
"""
return ((ipds+PI/2)*NUM_CLASSES/PI).long() # assumes input is tensor
def continuise(ipd_indices): # convert indices back to IPD midpoints
"""
Undo the discretisation of ipds
Parameters:
ipd_indices (array)
Returns:
array
"""
return (ipd_indices+0.5)/NUM_CLASSES*PI-PI/2
# Generator function iterates over the data in batches
# We randomly permute the order of the data to improve learning
def data_generator(ipds, spikes):
"""
Generate the whole training data by iterating over the data in the batches.
Order of the data is randomly permuted to improve learning.
Parameters:
ipds (tensor)
spikes (tensor): flag on the use of usual or null phase delays
Yields:
x_local (tensor): relates to the spikes
y_local (tensor): relates to the ipds
"""
perm = torch.randperm(spikes.shape[0])
spikes = spikes[perm, :, :]
ipds = ipds[perm]
n, _, _ = spikes.shape
n_batch = n//BATCH_SIZE
for i in range(n_batch):
x_local = spikes[i*BATCH_SIZE:(i+1)*BATCH_SIZE, :, :]
y_local = ipds[i*BATCH_SIZE:(i+1)*BATCH_SIZE]
yield x_local, y_local
# Plot a few just to show how it looks
def plot_some_input_examples(num_examples=8):
"""
Plots some example inputs.
Parameters:
num_examples (int): default = 8
"""
ipd, spikes = random_ipd_input_signal(num_examples)
spikes = spikes.cpu()
plt.figure(figsize=(10, 4), dpi=100)
for i in range(num_examples):
plt.subplot(2, 4, i+1)
plt.imshow(spikes[i, :, :].T, aspect='auto', interpolation='nearest', cmap=plt.cm.gray_r)
plt.title(f'True IPD = {int(ipd[i]*180/PI)} deg')
if i>=4:
plt.xlabel('Time (steps)')
if i%4==0:
plt.ylabel('Input neuron index')
plt.tight_layout()
plot_some_input_examples()
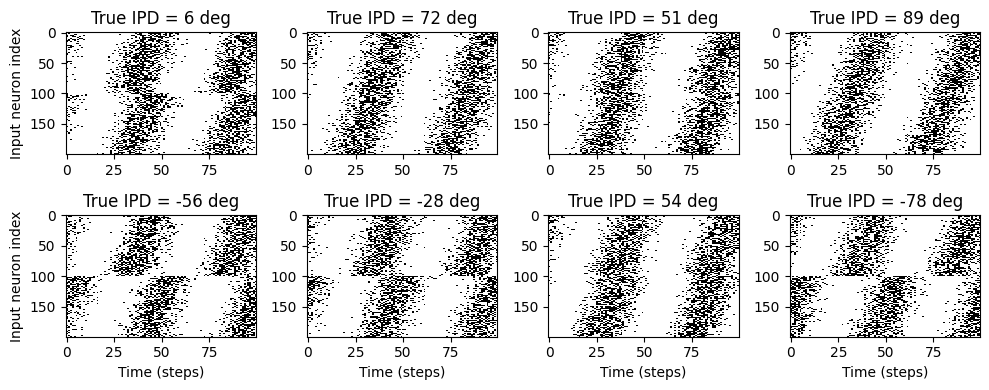
SNN¶
class SurrGradSpike(torch.autograd.Function):
@staticmethod
def forward(ctx, input):
ctx.save_for_backward(input)
out = torch.zeros_like(input)
out[input > 0] = 1.0
return out
@staticmethod
def backward(ctx, grad_output):
input, = ctx.saved_tensors
# Original SPyTorch/SuperSpike gradient
# This seems to be a typo or error? But it works well
#grad = grad_output/(100*torch.abs(input)+1.0)**2
# Sigmoid
grad = grad_output*BETA*torch.sigmoid(BETA*input)*(1-torch.sigmoid(BETA*input))
return grad
spike_fn = SurrGradSpike.apply
# Run the simulation
def snn(presynaptic_input_spikes_tensor, W1, W1_bis, W2,
multiple_connections_per_axon, single_neuron_model):
"""
Runs the SNN simulation.
Parameters:
presynaptic_input_spikes_tensor (tensor): corresponds to x_local in the training
W1 (tensor): initialised trainable weight parameter for the first layer
W2 (tensor): initialised trainable weight parameter for the second layer
multiple_connections_per_axon (boolean): flag on the use of multiple connections per axon,
single_neuron_model (function): a function that computes the output of a single neuron, given input over multiple segments
Returns:
v_rec (tensor): recorded membrane potential of output
input_smoothed (tensor): smoothed inputs
"""
# First layer: input to hidden
v = torch.zeros((BATCH_SIZE, NUM_HIDDEN), device=device, dtype=dtype)
s = torch.zeros((BATCH_SIZE, NUM_HIDDEN), device=device, dtype=dtype)
s_rec = [s]
cur_connections_per_axon = CONNECTIONS_PER_AXON if multiple_connections_per_axon else 1
inputs_for_all_segments_of_all_hidden = (presynaptic_input_spikes_tensor @ W1)
# BATCH_SIZE, DURATION_STEPS, NUM_HIDDEN * cur_connections_per_axon
inputs_for_all_segments_of_all_hidden = inputs_for_all_segments_of_all_hidden.transpose(1,2)
# BATCH_SIZE, NUM_HIDDEN * cur_connections_per_axon, DURATION_STEPS
inputs_for_all_segments_of_all_hidden_for_single_neuron = inputs_for_all_segments_of_all_hidden.reshape(BATCH_SIZE * NUM_HIDDEN, cur_connections_per_axon, DURATION_STEPS)
# BATCH_SIZE * NUM_HIDDEN, cur_connections_per_axon, DURATION_STEPS
# we use a handy trick here, enlarging the batch size, so we can use a single neuron model for all the neurons in the hidden layer
hidden_output = single_neuron_model(inputs_for_all_segments_of_all_hidden_for_single_neuron)
# BATCH_SIZE * NUM_HIDDEN, DURATION_STEPS
hidden_output = hidden_output.reshape(BATCH_SIZE, NUM_HIDDEN, DURATION_STEPS)
s_rec = hidden_output.transpose(1,2)
# Second layer: hidden to output
v = torch.zeros((BATCH_SIZE, NUM_CLASSES), device=device, dtype=dtype)
s = torch.zeros((BATCH_SIZE, NUM_CLASSES), device=device, dtype=dtype)
v_rec = [v]
h = torch.einsum("abc,cd->abd", (s_rec, W2))
alpha = np.exp(-DT/TAU)
for t in range(DURATION_STEPS - 1):
v = alpha*v + h[:, t, :]
v_rec.append(v)
v_rec = torch.stack(v_rec, dim=1)
# Return recorded membrane potential of output and smoothed input (for visualisation)
return v_rec
Training¶
# Weights and uniform weight initialisation
def init_weight_matrix():
"""
Initialises the weight matrix used in the fanin-fanout calculations for the initialisation of W1 and W2.
Parameters:
None
Returns:
W (tensor): weight tensor used in fanin-fanout calculations
"""
W = nn.Parameter(torch.empty((INPUT_SIZE, NUM_CLASSES), device=device, dtype=dtype, requires_grad=True))
fan_in, _ = nn.init._calculate_fan_in_and_fan_out(W)
bound = 1 / np.sqrt(fan_in)
nn.init.uniform_(W, -bound, bound)
return W
def init_weight_matrices(W, multiple_connections_per_axon=False):
"""
Initialises the weight matrices in the SNN: W1, W1_bis and W2.
Parameters:
W (tensor): weight tensor used in fanin-fanout calculations for W1 and W2
multiple_connections_per_axon (boolean): flag on the use of multiple connections per axon
Returns:
W1 (tensor): initialised trainable weight parameter for the first layer
W1_bis (tensor): fixed non-trainable weight parameter for the first layer when there are multiple connections per axon
W2 (tensor): initialised trainable weight parameter for the second layer
"""
if multiple_connections_per_axon: # Exp. 3
# Input to hidden layer
W1 = nn.Parameter(torch.empty((INPUT_SIZE, NUM_HIDDEN*CONNECTIONS_PER_AXON), device=device, dtype=dtype, requires_grad=True))
fan_in, _ = nn.init._calculate_fan_in_and_fan_out(W)
bound = 1 / np.sqrt(fan_in)
nn.init.uniform_(W1, -bound, bound)
# placeholder for W1_bis (not used)
W1_bis = torch.zeros(NUM_HIDDEN*CONNECTIONS_PER_AXON, NUM_HIDDEN)
# Hidden layer to output
W2 = nn.Parameter(torch.empty((NUM_HIDDEN, NUM_CLASSES), device=device, dtype=dtype, requires_grad=True))
fan_in, _ = nn.init._calculate_fan_in_and_fan_out(W)
bound = 1 / np.sqrt(fan_in)
nn.init.uniform_(W2, -bound, bound)
else:
# Input to hidden layer
W1 = nn.Parameter(torch.empty((INPUT_SIZE, NUM_HIDDEN), device=device, dtype=dtype, requires_grad=True))
fan_in, _ = nn.init._calculate_fan_in_and_fan_out(W)
bound = 1 / np.sqrt(fan_in)
nn.init.uniform_(W1, -bound, bound)
# placeholder for W1_bis (not used)
W1_bis = torch.zeros(NUM_HIDDEN*CONNECTIONS_PER_AXON, NUM_HIDDEN)
# Hidden layer to output
W2 = nn.Parameter(torch.empty((NUM_HIDDEN, NUM_CLASSES), device=device, dtype=dtype, requires_grad=True))
fan_in, _ = nn.init._calculate_fan_in_and_fan_out(W)
bound = 1 / np.sqrt(fan_in)
nn.init.uniform_(W2, -bound, bound)
return W1, W1_bis, W2
def train(ipds, spikes, usual_phase_delays=True, multiple_connections_per_axon=False, random_tau_constants=False, minimal_smoothing=False):
"""
Runs the training of the SNN simulation.
Parameters:
usual_phase_delays (boolean): flag on the use of usual or null phase delays
multiple_connections_per_axon (boolean): flag on the use of multiple connections per axon
random_tau_constants (boolean): flag on the use of randomly sampled tau hyperparameters (rise and decay)
minimal_smoothing (boolean): flag on the use of fast tau hyperparameters for minimal input spike smoothing
Returns:
ipds (tensor): training data (y)
spikes (tensor): training data (X)
W1 (tensor): trained W1
W2 (tensor): trained W2
snn_training_snapshot (list): snapshots of the training at each epoch
"""
# Generate the training data
#ipds, spikes = random_ipd_input_signal(NUM_SAMPLES, usual_phase_delays)
# Initialise weight matrices
W = init_weight_matrix() # for fan_in/out calculations
W1, W1_bis, W2 = init_weight_matrices(W, multiple_connections_per_axon)
# Optimiser and loss function
optimizer = torch.optim.Adam([W1, W2], lr=LR)
log_softmax_fn = nn.LogSoftmax(dim=1)
loss_fn = nn.NLLLoss()
loss_hist = []
snn_training_snapshot = []
# TODO: plug all of these parameters inside: random_tau_constants, minimal_smoothing, currently they are being ignored
single_neuron_model = FilterAndFireNeuron(count_segments=CONNECTIONS_PER_AXON if multiple_connections_per_axon else 1)
for e in pbar(range(NUM_EPOCHS)):
local_loss = []
batch_number = 0
print('--------------------------------------------------------')
print("EPOCH {}".format(e+1))
for x_local, y_local in data_generator(discretise(ipds), spikes):
batch_number += 1
# Run network
output = snn(x_local, W1, W1_bis, W2, multiple_connections_per_axon, single_neuron_model)
# Compute cross entropy loss
#m = torch.sum(output, 1)*0.01 # Sum time dimension
# Compute cross entropy loss
m = torch.mean(output, 1) # Mean across time dimension
reg = 0 # to add regularisation later if wanted
loss = loss_fn(log_softmax_fn(m), y_local) + reg
local_loss.append(loss.item())
# Update gradients
optimizer.zero_grad()
loss.backward()
optimizer.step()
# Record and print the loss of the current epoch
loss_hist.append(np.mean(local_loss))
print("---EPOCH %i: LOSS=%.5f"%(e+1, np.mean(local_loss)))
# Plot raster plot:
# e.g. input_smoothed for the 1st example of the last batch of the current epoch
#print("---Raster Plots at Epoch {} - Example #1/64 of the Last Batch Group".format(e+1))
#plt.figure(1)
#plt.title("input_smoothed example vs corresponding initial spike train")
#plt.subplot(211)
#plt.imshow(input_smoothed[0, :, :].detach().numpy().T, aspect='auto', interpolation='nearest', cmap=plt.cm.gray_r)
#plt.xlabel('Time (steps)')
#plt.ylabel('Input neuron index')
#plt.subplot(212)
#plt.imshow(x_local[0, :, :].detach().numpy().T, aspect='auto', interpolation='nearest', cmap=plt.cm.gray_r)
#plt.xlabel('Time (steps)')
#plt.ylabel('Input neuron index')
#plt.show()
# Take a snapshot of the model at the end of the current epoch.
## Use cases:
### if we want to resume training from this current epoch,
### or if the training is halted before the last epoch
snn_training_snapshot.append({'W1':W1, 'W2':W2,
'multiple_connections_per_axon':multiple_connections_per_axon,
'random_tau_constants':random_tau_constants,
'minimal_smoothing':minimal_smoothing})
# Plot the loss function over time
plt.plot(loss_hist)
plt.xlabel('Epoch')
plt.ylabel('Loss')
plt.tight_layout()
# return the train dataset (ipds, spikes) and trained weights for analysis
# also return the list of model snapshots for each epoch (in case we want to resume training)
return W1, W1_bis, W2, snn_training_snapshot, single_neuron_model
Train and Test Performance of the Trained Model¶
def get_accuracy(ipds, spikes, run):
"""
Gets the accuracy on data (train or test)
Parameters:
ipds (tensor)
spikes (tensor)
run (lambda function)
Returns:
ipd_true (list)
ipd_est (list)
confusion (numpy array)
accs (list)
"""
accs = []
ipd_true = []
ipd_est = []
confusion = np.zeros((NUM_CLASSES, NUM_CLASSES))
#print(ipds.shape)
#print(spikes.shape)
for x_local, y_local in data_generator(ipds, spikes):
y_local_orig = y_local
y_local = discretise(y_local)
output = run(x_local)
#m = torch.sum(output, 1) # Sum time dimension
m = torch.mean(output, 1)
_, am = torch.max(m, 1) # argmax over output units
tmp = np.mean((y_local == am).detach().cpu().numpy()) # compare to labels
for i, j in zip(y_local.detach().cpu().numpy(), am.detach().cpu().numpy()):
confusion[j, i] += 1
ipd_true.append(y_local_orig.cpu().data.numpy())
ipd_est.append(continuise(am.detach().cpu().numpy()))
accs.append(tmp)
ipd_true = np.hstack(ipd_true)
ipd_est = np.hstack(ipd_est)
return ipd_true, ipd_est, confusion, accs
def report_accuracy(ipd_true, ipd_est, confusion, accs, label):
"""
Plots the accuracy on data (train or test).
Parameters:
ipd_true (list)
ipd_est (list)
confusion (numpy array)
accs (list)
label (string): "Test" or "Train"
Returns:
None
"""
abs_errors_deg = abs(ipd_true-ipd_est)*180/PI
print()
print(f"{label} classifier accuracy: {100*np.mean(accs):.1f}%")
print(f"{label} absolute error: {np.mean(abs_errors_deg):.1f} deg")
plt.figure(figsize=(10, 4), dpi=100)
plt.subplot(121)
plt.hist(ipd_true * 180 / PI, bins=NUM_CLASSES, label='True')
plt.hist(ipd_est * 180 / PI, bins=NUM_CLASSES, label='Estimated')
plt.xlabel("IPD")
plt.yticks([])
plt.legend(loc='best')
plt.title(label)
plt.subplot(122)
confusion /= np.sum(confusion, axis=0)[np.newaxis, :]
plt.imshow(confusion, interpolation='nearest', aspect='equal', origin='lower', extent=(-90, 90, -90, 90))
plt.xlabel('True IPD')
plt.ylabel('Estimated IPD')
plt.title('Confusion matrix')
plt.tight_layout()
def analyse_accuracy(ipds, spikes, W1_trained, W1_bis, W2_trained, multiple_connections_per_axon, single_neuron_model, test_data=False):
"""
Analyses the accuracy on data (train or test)
Parameters:
ipds_train (tensor)
spikes_train (tensor)
W1_trained (tensor): trained W1
W2_trained (tensor): trained W2
multiple_connections_per_axon (boolean): flag on the use of multiple connections per axon
random_tau_constants (boolean): flag on the use of randomly sampled tau hyperparameters (rise and decay)
minimal_smoothing (boolean): flag on the use of fast tau hyperparameters for minimal input spike smoothing
test_data (boolean): flag on the use of test data for the analysis of the accuracy
Returns:
ipd_true (list)
ipd_est (list)
confusion (numpy array)
accs (list)
"""
run_function = lambda x: snn(x, W1_trained, W1_bis, W2_trained, multiple_connections_per_axon, single_neuron_model)
ipd_true, ipd_est, confusion, accs = get_accuracy(ipds, spikes, run_function)
# Analyse test accuracy
if test_data:
label = "Test"
# Analyse train accuracy
else:
label = "Train"
report_accuracy(ipd_true, ipd_est, confusion, accs, label)
return 100*np.mean(accs)
Alternative Filter-and-Fire Neuron Model¶
def create_psp_filter(temporal_length, tau_rise, tau_decay):
safety_factor = 1.5
if tau_rise >= (tau_decay / safety_factor):
tau_decay = safety_factor * tau_rise
exp_r = signal.exponential(temporal_length, 0, tau_rise, sym=False)
exp_d = signal.exponential(temporal_length, 0, tau_decay, sym=False)
post_syn_potential = exp_d - exp_r
post_syn_potential = post_syn_potential / np.max(post_syn_potential)
post_syn_potential = np.flipud(post_syn_potential)
return post_syn_potential
class PostSynapticPotentials(nn.Module):
def __init__(self, tau_rise_vec, tau_decay_vec):
super().__init__()
self.count_filters = len(tau_rise_vec)
self.tau_rise_vec = tau_rise_vec
self.tau_decay_vec = tau_decay_vec
self.temporal_filter_length = int(4 * np.max(tau_decay_vec)) + 1
temporal_filters = torch.zeros(self.count_filters, self.temporal_filter_length)
for i in range(self.count_filters):
temporal_filters[i, :] = torch.tensor(
create_psp_filter(self.temporal_filter_length, tau_rise_vec[i],
tau_decay_vec[i]).copy(), dtype=torch.float)
self.temporal_filters = nn.Parameter(temporal_filters, requires_grad=False)
def forward(self, x):
# 0 batch size, 1 channel_shape, 2 time
batch_size = x.shape[0]
x_time = x.shape[-1]
x_channel_shape = tuple(x.shape[1:-1])
x_channel_dim = np.prod(x_channel_shape)
if len(x_channel_shape) > 1:
# TODO: implement one day
raise ValueError('The input tensor must be 3 dimensional.')
if x_channel_dim != self.count_filters:
raise ValueError('The number of channels in the input tensor does not match the number of filters.')
x = x.reshape(-1, x_channel_dim, x.shape[-1])
x = torch.concat([torch.zeros(x.shape[0], x.shape[1], self.temporal_filter_length).to(x.device), x], dim=2)
filtered = torch.nn.functional.conv1d(x.float(), self.temporal_filters.unsqueeze(1).float(), padding=self.temporal_filter_length, groups=self.count_filters)[:,:,self.temporal_filter_length+1:-self.temporal_filter_length]
filtered = filtered.reshape(batch_size, x_channel_dim, x_time)
return filtered
class FilterAndFireNeuron(nn.Module):
def __init__(self, count_segments=5, tau_rise_range=[1, 16], tau_decay_range=[8, 30],
sort_tau_rise=False, sort_tau_decay=False, tau_rise_vec=None, tau_decay_vec=None,
return_currents=False, return_voltage=False):
super().__init__()
self.count_axons = 1
if tau_rise_vec is not None and tau_decay_vec is not None:
if len(tau_rise_vec) != len(tau_decay_vec):
raise ValueError('tau_rise_vec and tau_decay_vec must have the same length.')
self.count_segments = len(tau_rise_vec) // self.count_axons
self.effective_tau_rise_vec = tau_rise_vec
self.effective_tau_decay_vec = tau_decay_vec
self.psps = PostSynapticPotentials(tau_rise_vec, tau_decay_vec)
self.count_synapses = self.count_axons * self.count_segments
elif tau_rise_range is not None and tau_decay_range is not None and count_segments is not None:
self.count_segments = count_segments
self.count_synapses = self.count_axons * self.count_segments
# repeat the same filter for each axon
tau_rise_vec = np.random.uniform(tau_rise_range[0], tau_rise_range[1], self.count_synapses)
if sort_tau_rise:
# sort the tau_rise_vec to make sure that the first element is the largest
tau_rise_vec = np.sort(tau_rise_vec)[::-1]
tau_decay_vec = np.random.uniform(tau_decay_range[0], tau_decay_range[1], self.count_synapses)
if sort_tau_decay:
# sort the tau_decay_vec to make sure that the first element is the largest
tau_decay_vec = np.sort(tau_decay_vec)[::-1]
self.effective_tau_rise_vec = tau_rise_vec
self.effective_tau_decay_vec = tau_decay_vec
self.psps = PostSynapticPotentials(self.effective_tau_rise_vec, self.effective_tau_decay_vec)
else:
raise ValueError('Either tau_rise_vec and tau_decay_vec or tau_rise_range and tau_decay_range and count_segments must be specified.')
self.return_currents = return_currents
self.return_voltage = return_voltage
def forward(self, x):
batch_size = x.shape[0]
x_time = x.shape[-1]
x_channel_shape = tuple(x.shape[1:-1])
x_channel_dim = np.prod(x_channel_shape)
x = x.reshape(batch_size, x_channel_dim, x_time)
if x_channel_dim != self.count_segments:
raise ValueError('The number of channels does not match the number of segments.')
currents = self.psps(x)
if self.return_currents:
return currents
# sum over synapses
somatic_current = torch.sum(currents, dim=1)
# a naive approach for now (might want to have a resting membrane potential and a resistance)
somatic_voltage = somatic_current
v = torch.zeros(somatic_voltage.shape, device=device, dtype=dtype)
s = torch.zeros(somatic_voltage.shape, device=device, dtype=dtype)
alpha = np.exp(-DT/TAU)
for t in range(DURATION_STEPS - 1):
v[:, t+1] = (alpha*v[:, t] + somatic_voltage[:, t])*(1-s[:, t]) # multiply by 0 after a spike (similar to soma_current)
s[:, t+1] = spike_fn(v[:, t+1]-1) # threshold of 1
if self.return_voltage:
return v
return s
def get_model_shape(self):
return ((self.count_segments,), True, (1,), 0, 0.5)
Note: if the network performs at chance, the accuracy should be 1 out of 12 (since there are 12 classes).
Exp 1. Training & Testing Results¶
Using no multiple connections (M=1)¶
# training
# Generate the training data
ipds_train, spikes_train = random_ipd_input_signal(NUM_SAMPLES, usual_phase_delays=True)
Exp1_W1_trained, W1_bis, Exp1_W2_trained, Exp1_snn_training_snapshot, single_neuron_model = train(ipds_train, spikes_train,
multiple_connections_per_axon=False)
0%| | 0/100 [00:00<?, ?it/s]
--------------------------------------------------------
EPOCH 1
1%| | 1/100 [00:06<10:45, 6.52s/it]
---EPOCH 1: LOSS=2.80819
--------------------------------------------------------
EPOCH 2
2%|▏ | 2/100 [00:13<10:42, 6.55s/it]
---EPOCH 2: LOSS=2.56571
--------------------------------------------------------
EPOCH 3
3%|▎ | 3/100 [00:19<10:44, 6.65s/it]
---EPOCH 3: LOSS=2.46367
--------------------------------------------------------
EPOCH 4
4%|▍ | 4/100 [00:27<10:59, 6.87s/it]
---EPOCH 4: LOSS=2.39239
--------------------------------------------------------
EPOCH 5
5%|▌ | 5/100 [00:34<10:55, 6.90s/it]
---EPOCH 5: LOSS=2.34705
--------------------------------------------------------
EPOCH 6
6%|▌ | 6/100 [00:40<10:35, 6.76s/it]
---EPOCH 6: LOSS=2.30928
--------------------------------------------------------
EPOCH 7
7%|▋ | 7/100 [00:47<10:28, 6.76s/it]
---EPOCH 7: LOSS=2.27508
--------------------------------------------------------
EPOCH 8
8%|▊ | 8/100 [00:53<10:11, 6.64s/it]
---EPOCH 8: LOSS=2.24177
--------------------------------------------------------
EPOCH 9
9%|▉ | 9/100 [01:00<10:11, 6.72s/it]
---EPOCH 9: LOSS=2.21162
--------------------------------------------------------
EPOCH 10
10%|█ | 10/100 [01:08<10:30, 7.01s/it]
---EPOCH 10: LOSS=2.18304
--------------------------------------------------------
EPOCH 11
11%|█ | 11/100 [01:15<10:20, 6.98s/it]
---EPOCH 11: LOSS=2.15678
--------------------------------------------------------
EPOCH 12
12%|█▏ | 12/100 [01:22<10:14, 6.98s/it]
---EPOCH 12: LOSS=2.13329
--------------------------------------------------------
EPOCH 13
13%|█▎ | 13/100 [01:29<10:08, 6.99s/it]
---EPOCH 13: LOSS=2.11015
--------------------------------------------------------
EPOCH 14
14%|█▍ | 14/100 [01:35<09:54, 6.91s/it]
---EPOCH 14: LOSS=2.08944
--------------------------------------------------------
EPOCH 15
15%|█▌ | 15/100 [01:43<10:10, 7.18s/it]
---EPOCH 15: LOSS=2.06968
--------------------------------------------------------
EPOCH 16
16%|█▌ | 16/100 [01:50<10:00, 7.15s/it]
---EPOCH 16: LOSS=2.05117
--------------------------------------------------------
EPOCH 17
17%|█▋ | 17/100 [01:57<09:46, 7.07s/it]
---EPOCH 17: LOSS=2.03224
--------------------------------------------------------
EPOCH 18
18%|█▊ | 18/100 [02:04<09:34, 7.00s/it]
---EPOCH 18: LOSS=2.01423
--------------------------------------------------------
EPOCH 19
19%|█▉ | 19/100 [02:11<09:24, 6.97s/it]
---EPOCH 19: LOSS=1.99642
--------------------------------------------------------
EPOCH 20
20%|██ | 20/100 [02:18<09:18, 6.99s/it]
---EPOCH 20: LOSS=1.97965
--------------------------------------------------------
EPOCH 21
21%|██ | 21/100 [02:25<09:07, 6.94s/it]
---EPOCH 21: LOSS=1.96248
--------------------------------------------------------
EPOCH 22
22%|██▏ | 22/100 [02:32<08:59, 6.92s/it]
---EPOCH 22: LOSS=1.94670
--------------------------------------------------------
EPOCH 23
23%|██▎ | 23/100 [02:39<09:00, 7.02s/it]
---EPOCH 23: LOSS=1.93074
--------------------------------------------------------
EPOCH 24
24%|██▍ | 24/100 [02:46<09:07, 7.20s/it]
---EPOCH 24: LOSS=1.91624
--------------------------------------------------------
EPOCH 25
25%|██▌ | 25/100 [02:53<08:55, 7.15s/it]
---EPOCH 25: LOSS=1.90246
--------------------------------------------------------
EPOCH 26
26%|██▌ | 26/100 [03:00<08:45, 7.10s/it]
---EPOCH 26: LOSS=1.88736
--------------------------------------------------------
EPOCH 27
27%|██▋ | 27/100 [03:08<08:38, 7.10s/it]
---EPOCH 27: LOSS=1.87312
--------------------------------------------------------
EPOCH 28
28%|██▊ | 28/100 [03:15<08:31, 7.10s/it]
---EPOCH 28: LOSS=1.85814
--------------------------------------------------------
EPOCH 29
29%|██▉ | 29/100 [03:22<08:32, 7.22s/it]
---EPOCH 29: LOSS=1.84455
--------------------------------------------------------
EPOCH 30
30%|███ | 30/100 [03:29<08:14, 7.07s/it]
---EPOCH 30: LOSS=1.83083
--------------------------------------------------------
EPOCH 31
31%|███ | 31/100 [03:36<07:59, 6.95s/it]
---EPOCH 31: LOSS=1.81682
--------------------------------------------------------
EPOCH 32
32%|███▏ | 32/100 [03:43<07:58, 7.03s/it]
---EPOCH 32: LOSS=1.80451
--------------------------------------------------------
EPOCH 33
33%|███▎ | 33/100 [03:50<07:54, 7.09s/it]
---EPOCH 33: LOSS=1.79022
--------------------------------------------------------
EPOCH 34
34%|███▍ | 34/100 [03:57<07:45, 7.05s/it]
---EPOCH 34: LOSS=1.77817
--------------------------------------------------------
EPOCH 35
35%|███▌ | 35/100 [04:04<07:37, 7.04s/it]
---EPOCH 35: LOSS=1.76454
--------------------------------------------------------
EPOCH 36
36%|███▌ | 36/100 [04:11<07:28, 7.01s/it]
---EPOCH 36: LOSS=1.75081
--------------------------------------------------------
EPOCH 37
37%|███▋ | 37/100 [04:19<07:34, 7.22s/it]
---EPOCH 37: LOSS=1.73887
--------------------------------------------------------
EPOCH 38
38%|███▊ | 38/100 [04:25<07:21, 7.12s/it]
---EPOCH 38: LOSS=1.72622
--------------------------------------------------------
EPOCH 39
39%|███▉ | 39/100 [04:32<07:09, 7.05s/it]
---EPOCH 39: LOSS=1.71504
--------------------------------------------------------
EPOCH 40
40%|████ | 40/100 [04:40<07:06, 7.12s/it]
---EPOCH 40: LOSS=1.70258
--------------------------------------------------------
EPOCH 41
41%|████ | 41/100 [04:46<06:50, 6.96s/it]
---EPOCH 41: LOSS=1.69242
--------------------------------------------------------
EPOCH 42
42%|████▏ | 42/100 [04:54<06:49, 7.06s/it]
---EPOCH 42: LOSS=1.68099
--------------------------------------------------------
EPOCH 43
43%|████▎ | 43/100 [05:00<06:38, 6.99s/it]
---EPOCH 43: LOSS=1.66983
--------------------------------------------------------
EPOCH 44
44%|████▍ | 44/100 [05:07<06:29, 6.96s/it]
---EPOCH 44: LOSS=1.65832
--------------------------------------------------------
EPOCH 45
45%|████▌ | 45/100 [05:14<06:21, 6.94s/it]
---EPOCH 45: LOSS=1.64753
--------------------------------------------------------
EPOCH 46
46%|████▌ | 46/100 [05:21<06:12, 6.90s/it]
---EPOCH 46: LOSS=1.63689
--------------------------------------------------------
EPOCH 47
47%|████▋ | 47/100 [05:28<06:05, 6.89s/it]
---EPOCH 47: LOSS=1.62782
--------------------------------------------------------
EPOCH 48
48%|████▊ | 48/100 [05:34<05:53, 6.79s/it]
---EPOCH 48: LOSS=1.61655
--------------------------------------------------------
EPOCH 49
49%|████▉ | 49/100 [05:42<05:53, 6.94s/it]
---EPOCH 49: LOSS=1.60632
--------------------------------------------------------
EPOCH 50
50%|█████ | 50/100 [05:49<05:46, 6.92s/it]
---EPOCH 50: LOSS=1.59681
--------------------------------------------------------
EPOCH 51
51%|█████ | 51/100 [05:55<05:37, 6.89s/it]
---EPOCH 51: LOSS=1.58720
--------------------------------------------------------
EPOCH 52
52%|█████▏ | 52/100 [06:02<05:27, 6.83s/it]
---EPOCH 52: LOSS=1.57817
--------------------------------------------------------
EPOCH 53
53%|█████▎ | 53/100 [06:09<05:17, 6.75s/it]
---EPOCH 53: LOSS=1.56839
--------------------------------------------------------
EPOCH 54
54%|█████▍ | 54/100 [06:15<05:06, 6.67s/it]
---EPOCH 54: LOSS=1.55952
--------------------------------------------------------
EPOCH 55
55%|█████▌ | 55/100 [06:21<04:56, 6.58s/it]
---EPOCH 55: LOSS=1.55179
--------------------------------------------------------
EPOCH 56
56%|█████▌ | 56/100 [06:28<04:49, 6.58s/it]
---EPOCH 56: LOSS=1.54211
--------------------------------------------------------
EPOCH 57
57%|█████▋ | 57/100 [06:35<04:42, 6.56s/it]
---EPOCH 57: LOSS=1.53133
--------------------------------------------------------
EPOCH 58
58%|█████▊ | 58/100 [06:41<04:35, 6.55s/it]
---EPOCH 58: LOSS=1.52345
--------------------------------------------------------
EPOCH 59
59%|█████▉ | 59/100 [06:48<04:27, 6.53s/it]
---EPOCH 59: LOSS=1.51453
--------------------------------------------------------
EPOCH 60
60%|██████ | 60/100 [06:54<04:18, 6.47s/it]
---EPOCH 60: LOSS=1.50648
--------------------------------------------------------
EPOCH 61
61%|██████ | 61/100 [07:01<04:14, 6.52s/it]
---EPOCH 61: LOSS=1.49838
--------------------------------------------------------
EPOCH 62
62%|██████▏ | 62/100 [07:07<04:07, 6.50s/it]
---EPOCH 62: LOSS=1.48960
--------------------------------------------------------
EPOCH 63
63%|██████▎ | 63/100 [07:14<04:01, 6.53s/it]
---EPOCH 63: LOSS=1.48082
--------------------------------------------------------
EPOCH 64
64%|██████▍ | 64/100 [07:21<03:59, 6.64s/it]
---EPOCH 64: LOSS=1.47425
--------------------------------------------------------
EPOCH 65
65%|██████▌ | 65/100 [07:28<04:00, 6.86s/it]
---EPOCH 65: LOSS=1.46543
--------------------------------------------------------
EPOCH 66
66%|██████▌ | 66/100 [07:35<03:58, 7.02s/it]
---EPOCH 66: LOSS=1.45702
--------------------------------------------------------
EPOCH 67
67%|██████▋ | 67/100 [07:43<03:54, 7.11s/it]
---EPOCH 67: LOSS=1.44927
--------------------------------------------------------
EPOCH 68
68%|██████▊ | 68/100 [07:49<03:41, 6.91s/it]
---EPOCH 68: LOSS=1.44185
--------------------------------------------------------
EPOCH 69
69%|██████▉ | 69/100 [07:55<03:28, 6.73s/it]
---EPOCH 69: LOSS=1.43385
--------------------------------------------------------
EPOCH 70
70%|███████ | 70/100 [08:02<03:21, 6.72s/it]
---EPOCH 70: LOSS=1.42711
--------------------------------------------------------
EPOCH 71
71%|███████ | 71/100 [08:09<03:13, 6.67s/it]
---EPOCH 71: LOSS=1.41897
--------------------------------------------------------
EPOCH 72
72%|███████▏ | 72/100 [08:16<03:12, 6.87s/it]
---EPOCH 72: LOSS=1.41135
--------------------------------------------------------
EPOCH 73
73%|███████▎ | 73/100 [08:24<03:11, 7.11s/it]
---EPOCH 73: LOSS=1.40472
--------------------------------------------------------
EPOCH 74
74%|███████▍ | 74/100 [08:30<03:02, 7.03s/it]
---EPOCH 74: LOSS=1.39800
--------------------------------------------------------
EPOCH 75
75%|███████▌ | 75/100 [08:37<02:52, 6.90s/it]
---EPOCH 75: LOSS=1.39094
--------------------------------------------------------
EPOCH 76
76%|███████▌ | 76/100 [08:43<02:38, 6.61s/it]
---EPOCH 76: LOSS=1.38453
--------------------------------------------------------
EPOCH 77
77%|███████▋ | 77/100 [08:50<02:34, 6.71s/it]
---EPOCH 77: LOSS=1.37715
--------------------------------------------------------
EPOCH 78
78%|███████▊ | 78/100 [08:56<02:24, 6.57s/it]
---EPOCH 78: LOSS=1.37000
--------------------------------------------------------
EPOCH 79
79%|███████▉ | 79/100 [09:02<02:12, 6.33s/it]
---EPOCH 79: LOSS=1.36330
--------------------------------------------------------
EPOCH 80
80%|████████ | 80/100 [09:07<02:00, 6.05s/it]
---EPOCH 80: LOSS=1.35700
--------------------------------------------------------
EPOCH 81
81%|████████ | 81/100 [09:14<01:59, 6.27s/it]
---EPOCH 81: LOSS=1.34961
--------------------------------------------------------
EPOCH 82
82%|████████▏ | 82/100 [09:21<01:57, 6.53s/it]
---EPOCH 82: LOSS=1.34357
--------------------------------------------------------
EPOCH 83
83%|████████▎ | 83/100 [09:28<01:52, 6.59s/it]
---EPOCH 83: LOSS=1.33732
--------------------------------------------------------
EPOCH 84
84%|████████▍ | 84/100 [09:35<01:45, 6.61s/it]
---EPOCH 84: LOSS=1.33051
--------------------------------------------------------
EPOCH 85
85%|████████▌ | 85/100 [09:41<01:39, 6.65s/it]
---EPOCH 85: LOSS=1.32431
--------------------------------------------------------
EPOCH 86
86%|████████▌ | 86/100 [09:48<01:32, 6.57s/it]
---EPOCH 86: LOSS=1.31801
--------------------------------------------------------
EPOCH 87
87%|████████▋ | 87/100 [09:55<01:26, 6.65s/it]
---EPOCH 87: LOSS=1.31209
--------------------------------------------------------
EPOCH 88
88%|████████▊ | 88/100 [10:02<01:23, 6.94s/it]
---EPOCH 88: LOSS=1.30424
--------------------------------------------------------
EPOCH 89
89%|████████▉ | 89/100 [10:09<01:15, 6.84s/it]
---EPOCH 89: LOSS=1.29828
--------------------------------------------------------
EPOCH 90
90%|█████████ | 90/100 [10:15<01:06, 6.70s/it]
---EPOCH 90: LOSS=1.29306
--------------------------------------------------------
EPOCH 91
91%|█████████ | 91/100 [10:22<00:59, 6.64s/it]
---EPOCH 91: LOSS=1.28684
--------------------------------------------------------
EPOCH 92
92%|█████████▏| 92/100 [10:29<00:53, 6.73s/it]
---EPOCH 92: LOSS=1.28089
--------------------------------------------------------
EPOCH 93
93%|█████████▎| 93/100 [10:36<00:47, 6.81s/it]
---EPOCH 93: LOSS=1.27578
--------------------------------------------------------
EPOCH 94
94%|█████████▍| 94/100 [10:43<00:41, 6.84s/it]
---EPOCH 94: LOSS=1.26922
--------------------------------------------------------
EPOCH 95
95%|█████████▌| 95/100 [10:49<00:33, 6.77s/it]
---EPOCH 95: LOSS=1.26398
--------------------------------------------------------
EPOCH 96
96%|█████████▌| 96/100 [10:56<00:27, 6.81s/it]
---EPOCH 96: LOSS=1.25886
--------------------------------------------------------
EPOCH 97
97%|█████████▋| 97/100 [11:02<00:19, 6.67s/it]
---EPOCH 97: LOSS=1.25203
--------------------------------------------------------
EPOCH 98
98%|█████████▊| 98/100 [11:09<00:13, 6.60s/it]
---EPOCH 98: LOSS=1.24684
--------------------------------------------------------
EPOCH 99
99%|█████████▉| 99/100 [11:15<00:06, 6.51s/it]
---EPOCH 99: LOSS=1.24116
--------------------------------------------------------
EPOCH 100
100%|██████████| 100/100 [11:21<00:00, 6.82s/it]
---EPOCH 100: LOSS=1.23610
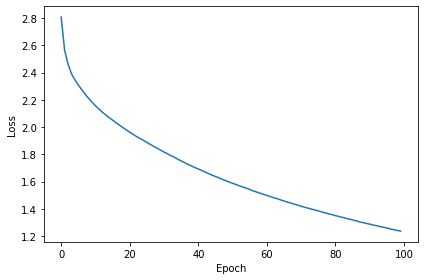
# training and testing accuracies
Exp1Fast_train_accuracy = analyse_accuracy(ipds_train, spikes_train, Exp1_W1_trained, W1_bis, Exp1_W2_trained,
multiple_connections_per_axon=False, single_neuron_model=single_neuron_model,
test_data=False)
# Generate the test data
ipds_test, spikes_test = random_ipd_input_signal(BATCH_SIZE*N_TESTING_BATCHES, usual_phase_delays=True)
Exp1_test_accuracy = analyse_accuracy(ipds_test, spikes_test, Exp1_W1_trained, W1_bis, Exp1_W2_trained,
multiple_connections_per_axon=False, single_neuron_model=single_neuron_model,
test_data=True)
Train classifier accuracy: 63.3%
Train absolute error: 7.2 deg
Test classifier accuracy: 57.3%
Test absolute error: 7.8 deg
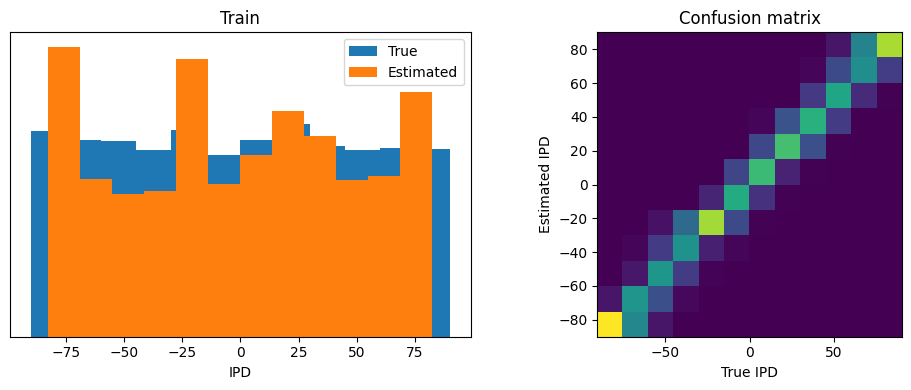
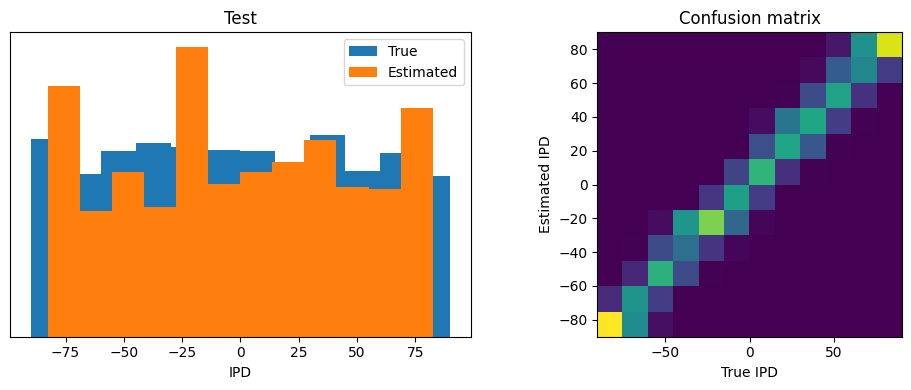
Exp 2. Training & Testing Results¶
Using multiple connections (M=3)¶
# training
# Generate the training data
ipds_train, spikes_train = random_ipd_input_signal(NUM_SAMPLES, usual_phase_delays=True)
Exp2_W1_trained, W1_bis, Exp2_W2_trained, Exp2_snn_training_snapshot, single_neuron_model = train(ipds_train, spikes_train,
multiple_connections_per_axon=True)
0%| | 0/100 [00:00<?, ?it/s]
--------------------------------------------------------
EPOCH 1
1%| | 1/100 [00:09<16:14, 9.85s/it]
---EPOCH 1: LOSS=2.77975
--------------------------------------------------------
EPOCH 2
2%|▏ | 2/100 [00:19<15:33, 9.53s/it]
---EPOCH 2: LOSS=2.48943
--------------------------------------------------------
EPOCH 3
3%|▎ | 3/100 [00:28<15:03, 9.31s/it]
---EPOCH 3: LOSS=2.43309
--------------------------------------------------------
EPOCH 4
4%|▍ | 4/100 [00:37<14:55, 9.33s/it]
---EPOCH 4: LOSS=2.39790
--------------------------------------------------------
EPOCH 5
5%|▌ | 5/100 [00:47<14:52, 9.39s/it]
---EPOCH 5: LOSS=2.36529
--------------------------------------------------------
EPOCH 6
6%|▌ | 6/100 [00:55<14:24, 9.20s/it]
---EPOCH 6: LOSS=2.33296
--------------------------------------------------------
EPOCH 7
7%|▋ | 7/100 [01:04<14:06, 9.10s/it]
---EPOCH 7: LOSS=2.30643
--------------------------------------------------------
EPOCH 8
8%|▊ | 8/100 [01:13<13:55, 9.09s/it]
---EPOCH 8: LOSS=2.28021
--------------------------------------------------------
EPOCH 9
9%|▉ | 9/100 [01:22<13:34, 8.95s/it]
---EPOCH 9: LOSS=2.25632
--------------------------------------------------------
EPOCH 10
10%|█ | 10/100 [01:31<13:14, 8.83s/it]
---EPOCH 10: LOSS=2.23011
--------------------------------------------------------
EPOCH 11
11%|█ | 11/100 [01:39<13:08, 8.86s/it]
---EPOCH 11: LOSS=2.20565
--------------------------------------------------------
EPOCH 12
12%|█▏ | 12/100 [01:49<13:10, 8.99s/it]
---EPOCH 12: LOSS=2.18536
--------------------------------------------------------
EPOCH 13
13%|█▎ | 13/100 [01:58<13:07, 9.05s/it]
---EPOCH 13: LOSS=2.15993
--------------------------------------------------------
EPOCH 14
14%|█▍ | 14/100 [02:08<13:13, 9.23s/it]
---EPOCH 14: LOSS=2.13865
--------------------------------------------------------
EPOCH 15
15%|█▌ | 15/100 [02:17<13:19, 9.40s/it]
---EPOCH 15: LOSS=2.11837
--------------------------------------------------------
EPOCH 16
16%|█▌ | 16/100 [02:27<13:15, 9.47s/it]
---EPOCH 16: LOSS=2.09663
--------------------------------------------------------
EPOCH 17
17%|█▋ | 17/100 [02:36<13:03, 9.44s/it]
---EPOCH 17: LOSS=2.07650
--------------------------------------------------------
EPOCH 18
18%|█▊ | 18/100 [02:46<12:52, 9.42s/it]
---EPOCH 18: LOSS=2.05805
--------------------------------------------------------
EPOCH 19
19%|█▉ | 19/100 [02:56<12:50, 9.52s/it]
---EPOCH 19: LOSS=2.03774
--------------------------------------------------------
EPOCH 20
20%|██ | 20/100 [03:05<12:40, 9.50s/it]
---EPOCH 20: LOSS=2.01751
--------------------------------------------------------
EPOCH 21
21%|██ | 21/100 [03:15<12:32, 9.52s/it]
---EPOCH 21: LOSS=1.99678
--------------------------------------------------------
EPOCH 22
22%|██▏ | 22/100 [03:24<12:32, 9.65s/it]
---EPOCH 22: LOSS=1.97186
--------------------------------------------------------
EPOCH 23
23%|██▎ | 23/100 [03:34<12:22, 9.64s/it]
---EPOCH 23: LOSS=1.95140
--------------------------------------------------------
EPOCH 24
24%|██▍ | 24/100 [03:43<11:51, 9.36s/it]
---EPOCH 24: LOSS=1.93431
--------------------------------------------------------
EPOCH 25
25%|██▌ | 25/100 [03:52<11:47, 9.43s/it]
---EPOCH 25: LOSS=1.91304
--------------------------------------------------------
EPOCH 26
26%|██▌ | 26/100 [04:02<11:42, 9.49s/it]
---EPOCH 26: LOSS=1.89475
--------------------------------------------------------
EPOCH 27
27%|██▋ | 27/100 [04:13<11:59, 9.86s/it]
---EPOCH 27: LOSS=1.87521
--------------------------------------------------------
EPOCH 28
28%|██▊ | 28/100 [04:23<11:49, 9.85s/it]
---EPOCH 28: LOSS=1.85788
--------------------------------------------------------
EPOCH 29
29%|██▉ | 29/100 [04:32<11:34, 9.78s/it]
---EPOCH 29: LOSS=1.84079
--------------------------------------------------------
EPOCH 30
30%|███ | 30/100 [04:41<11:08, 9.55s/it]
---EPOCH 30: LOSS=1.82494
--------------------------------------------------------
EPOCH 31
31%|███ | 31/100 [04:50<10:45, 9.36s/it]
---EPOCH 31: LOSS=1.80928
--------------------------------------------------------
EPOCH 32
32%|███▏ | 32/100 [04:59<10:24, 9.18s/it]
---EPOCH 32: LOSS=1.79311
--------------------------------------------------------
EPOCH 33
33%|███▎ | 33/100 [05:08<10:07, 9.07s/it]
---EPOCH 33: LOSS=1.77583
--------------------------------------------------------
EPOCH 34
34%|███▍ | 34/100 [05:17<09:57, 9.06s/it]
---EPOCH 34: LOSS=1.75982
--------------------------------------------------------
EPOCH 35
35%|███▌ | 35/100 [05:26<09:45, 9.00s/it]
---EPOCH 35: LOSS=1.74054
--------------------------------------------------------
EPOCH 36
36%|███▌ | 36/100 [05:34<09:29, 8.89s/it]
---EPOCH 36: LOSS=1.72292
--------------------------------------------------------
EPOCH 37
37%|███▋ | 37/100 [05:43<09:21, 8.91s/it]
---EPOCH 37: LOSS=1.70417
--------------------------------------------------------
EPOCH 38
38%|███▊ | 38/100 [05:52<09:10, 8.87s/it]
---EPOCH 38: LOSS=1.68707
--------------------------------------------------------
EPOCH 39
39%|███▉ | 39/100 [06:01<08:59, 8.85s/it]
---EPOCH 39: LOSS=1.67016
--------------------------------------------------------
EPOCH 40
40%|████ | 40/100 [06:10<09:03, 9.06s/it]
---EPOCH 40: LOSS=1.65586
--------------------------------------------------------
EPOCH 41
41%|████ | 41/100 [06:19<08:55, 9.07s/it]
---EPOCH 41: LOSS=1.64253
--------------------------------------------------------
EPOCH 42
42%|████▏ | 42/100 [06:28<08:45, 9.06s/it]
---EPOCH 42: LOSS=1.62788
--------------------------------------------------------
EPOCH 43
43%|████▎ | 43/100 [06:37<08:31, 8.97s/it]
---EPOCH 43: LOSS=1.61478
--------------------------------------------------------
EPOCH 44
44%|████▍ | 44/100 [06:46<08:20, 8.94s/it]
---EPOCH 44: LOSS=1.60126
--------------------------------------------------------
EPOCH 45
45%|████▌ | 45/100 [06:55<08:11, 8.94s/it]
---EPOCH 45: LOSS=1.59007
--------------------------------------------------------
EPOCH 46
46%|████▌ | 46/100 [07:04<08:00, 8.89s/it]
---EPOCH 46: LOSS=1.57802
--------------------------------------------------------
EPOCH 47
47%|████▋ | 47/100 [07:13<07:51, 8.90s/it]
---EPOCH 47: LOSS=1.56665
--------------------------------------------------------
EPOCH 48
48%|████▊ | 48/100 [07:22<07:40, 8.86s/it]
---EPOCH 48: LOSS=1.55513
--------------------------------------------------------
EPOCH 49
49%|████▉ | 49/100 [07:30<07:31, 8.85s/it]
---EPOCH 49: LOSS=1.54493
--------------------------------------------------------
EPOCH 50
50%|█████ | 50/100 [07:39<07:19, 8.79s/it]
---EPOCH 50: LOSS=1.53372
--------------------------------------------------------
EPOCH 51
51%|█████ | 51/100 [07:48<07:07, 8.72s/it]
---EPOCH 51: LOSS=1.52248
--------------------------------------------------------
EPOCH 52
52%|█████▏ | 52/100 [07:56<06:59, 8.75s/it]
---EPOCH 52: LOSS=1.51212
--------------------------------------------------------
EPOCH 53
53%|█████▎ | 53/100 [08:06<07:01, 8.97s/it]
---EPOCH 53: LOSS=1.50200
--------------------------------------------------------
EPOCH 54
54%|█████▍ | 54/100 [08:15<07:01, 9.17s/it]
---EPOCH 54: LOSS=1.49226
--------------------------------------------------------
EPOCH 55
55%|█████▌ | 55/100 [08:25<06:57, 9.28s/it]
---EPOCH 55: LOSS=1.48441
--------------------------------------------------------
EPOCH 56
56%|█████▌ | 56/100 [08:34<06:41, 9.13s/it]
---EPOCH 56: LOSS=1.47373
--------------------------------------------------------
EPOCH 57
57%|█████▋ | 57/100 [08:43<06:31, 9.11s/it]
---EPOCH 57: LOSS=1.46426
--------------------------------------------------------
EPOCH 58
58%|█████▊ | 58/100 [08:52<06:22, 9.11s/it]
---EPOCH 58: LOSS=1.45492
--------------------------------------------------------
EPOCH 59
59%|█████▉ | 59/100 [09:01<06:15, 9.15s/it]
---EPOCH 59: LOSS=1.44661
--------------------------------------------------------
EPOCH 60
60%|██████ | 60/100 [09:10<06:03, 9.09s/it]
---EPOCH 60: LOSS=1.43711
--------------------------------------------------------
EPOCH 61
61%|██████ | 61/100 [09:20<06:03, 9.31s/it]
---EPOCH 61: LOSS=1.42963
--------------------------------------------------------
EPOCH 62
62%|██████▏ | 62/100 [09:29<05:47, 9.15s/it]
---EPOCH 62: LOSS=1.42165
--------------------------------------------------------
EPOCH 63
63%|██████▎ | 63/100 [09:38<05:36, 9.09s/it]
---EPOCH 63: LOSS=1.41403
--------------------------------------------------------
EPOCH 64
64%|██████▍ | 64/100 [09:48<05:45, 9.60s/it]
---EPOCH 64: LOSS=1.40536
--------------------------------------------------------
EPOCH 65
65%|██████▌ | 65/100 [09:59<05:44, 9.85s/it]
---EPOCH 65: LOSS=1.39788
--------------------------------------------------------
EPOCH 66
66%|██████▌ | 66/100 [10:10<05:43, 10.11s/it]
---EPOCH 66: LOSS=1.39010
--------------------------------------------------------
EPOCH 67
67%|██████▋ | 67/100 [10:20<05:37, 10.24s/it]
---EPOCH 67: LOSS=1.38253
--------------------------------------------------------
EPOCH 68
68%|██████▊ | 68/100 [10:31<05:31, 10.37s/it]
---EPOCH 68: LOSS=1.37322
--------------------------------------------------------
EPOCH 69
69%|██████▉ | 69/100 [10:44<05:45, 11.15s/it]
---EPOCH 69: LOSS=1.36716
--------------------------------------------------------
EPOCH 70
70%|███████ | 70/100 [10:56<05:42, 11.40s/it]
---EPOCH 70: LOSS=1.35901
--------------------------------------------------------
EPOCH 71
71%|███████ | 71/100 [11:08<05:36, 11.60s/it]
---EPOCH 71: LOSS=1.35109
--------------------------------------------------------
EPOCH 72
72%|███████▏ | 72/100 [11:21<05:33, 11.92s/it]
---EPOCH 72: LOSS=1.34413
--------------------------------------------------------
EPOCH 73
73%|███████▎ | 73/100 [11:30<05:03, 11.24s/it]
---EPOCH 73: LOSS=1.33700
--------------------------------------------------------
EPOCH 74
74%|███████▍ | 74/100 [11:40<04:37, 10.69s/it]
---EPOCH 74: LOSS=1.33069
--------------------------------------------------------
EPOCH 75
75%|███████▌ | 75/100 [11:49<04:16, 10.28s/it]
---EPOCH 75: LOSS=1.32303
--------------------------------------------------------
EPOCH 76
76%|███████▌ | 76/100 [12:00<04:09, 10.41s/it]
---EPOCH 76: LOSS=1.31761
--------------------------------------------------------
EPOCH 77
77%|███████▋ | 77/100 [12:09<03:55, 10.22s/it]
---EPOCH 77: LOSS=1.30953
--------------------------------------------------------
EPOCH 78
78%|███████▊ | 78/100 [12:19<03:40, 10.04s/it]
---EPOCH 78: LOSS=1.30399
--------------------------------------------------------
EPOCH 79
79%|███████▉ | 79/100 [12:29<03:30, 10.01s/it]
---EPOCH 79: LOSS=1.29726
--------------------------------------------------------
EPOCH 80
80%|████████ | 80/100 [12:39<03:20, 10.04s/it]
---EPOCH 80: LOSS=1.29030
--------------------------------------------------------
EPOCH 81
81%|████████ | 81/100 [12:50<03:17, 10.39s/it]
---EPOCH 81: LOSS=1.28427
--------------------------------------------------------
EPOCH 82
82%|████████▏ | 82/100 [13:00<03:04, 10.25s/it]
---EPOCH 82: LOSS=1.27807
--------------------------------------------------------
EPOCH 83
83%|████████▎ | 83/100 [13:10<02:52, 10.13s/it]
---EPOCH 83: LOSS=1.27155
--------------------------------------------------------
EPOCH 84
84%|████████▍ | 84/100 [13:20<02:39, 9.96s/it]
---EPOCH 84: LOSS=1.26524
--------------------------------------------------------
EPOCH 85
85%|████████▌ | 85/100 [13:29<02:25, 9.73s/it]
---EPOCH 85: LOSS=1.26003
--------------------------------------------------------
EPOCH 86
86%|████████▌ | 86/100 [13:38<02:14, 9.59s/it]
---EPOCH 86: LOSS=1.25504
--------------------------------------------------------
EPOCH 87
87%|████████▋ | 87/100 [13:47<02:03, 9.53s/it]
---EPOCH 87: LOSS=1.24881
--------------------------------------------------------
EPOCH 88
88%|████████▊ | 88/100 [13:57<01:53, 9.46s/it]
---EPOCH 88: LOSS=1.24128
--------------------------------------------------------
EPOCH 89
89%|████████▉ | 89/100 [14:07<01:46, 9.65s/it]
---EPOCH 89: LOSS=1.23756
--------------------------------------------------------
EPOCH 90
90%|█████████ | 90/100 [14:18<01:40, 10.03s/it]
---EPOCH 90: LOSS=1.23236
--------------------------------------------------------
EPOCH 91
91%|█████████ | 91/100 [14:27<01:29, 9.91s/it]
---EPOCH 91: LOSS=1.22519
--------------------------------------------------------
EPOCH 92
92%|█████████▏| 92/100 [14:37<01:17, 9.73s/it]
---EPOCH 92: LOSS=1.22161
--------------------------------------------------------
EPOCH 93
93%|█████████▎| 93/100 [14:46<01:06, 9.51s/it]
---EPOCH 93: LOSS=1.21654
--------------------------------------------------------
EPOCH 94
94%|█████████▍| 94/100 [14:55<00:57, 9.54s/it]
---EPOCH 94: LOSS=1.21127
--------------------------------------------------------
EPOCH 95
95%|█████████▌| 95/100 [15:05<00:47, 9.45s/it]
---EPOCH 95: LOSS=1.20618
--------------------------------------------------------
EPOCH 96
96%|█████████▌| 96/100 [15:14<00:37, 9.45s/it]
---EPOCH 96: LOSS=1.20015
--------------------------------------------------------
EPOCH 97
97%|█████████▋| 97/100 [15:23<00:28, 9.38s/it]
---EPOCH 97: LOSS=1.19487
--------------------------------------------------------
EPOCH 98
98%|█████████▊| 98/100 [15:32<00:18, 9.31s/it]
---EPOCH 98: LOSS=1.19023
--------------------------------------------------------
EPOCH 99
99%|█████████▉| 99/100 [15:42<00:09, 9.33s/it]
---EPOCH 99: LOSS=1.18684
--------------------------------------------------------
EPOCH 100
100%|██████████| 100/100 [15:51<00:00, 9.52s/it]
---EPOCH 100: LOSS=1.18137
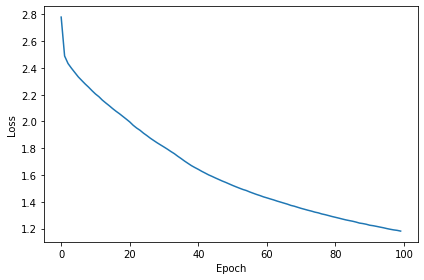
# training and testing accuracies
Exp2_train_accuracy = analyse_accuracy(ipds_train, spikes_train, Exp2_W1_trained, W1_bis, Exp2_W2_trained,
multiple_connections_per_axon=True, single_neuron_model=single_neuron_model,
test_data=False)
# Generate the test data
ipds_test, spikes_test = random_ipd_input_signal(BATCH_SIZE*N_TESTING_BATCHES, usual_phase_delays=True)
Exp2_test_accuracy = analyse_accuracy(ipds_test, spikes_test, Exp2_W1_trained, W1_bis, Exp2_W2_trained,
multiple_connections_per_axon=True, single_neuron_model=single_neuron_model,
test_data=True)
Train classifier accuracy: 65.6%
Train absolute error: 6.9 deg
Test classifier accuracy: 31.1%
Test absolute error: 16.9 deg
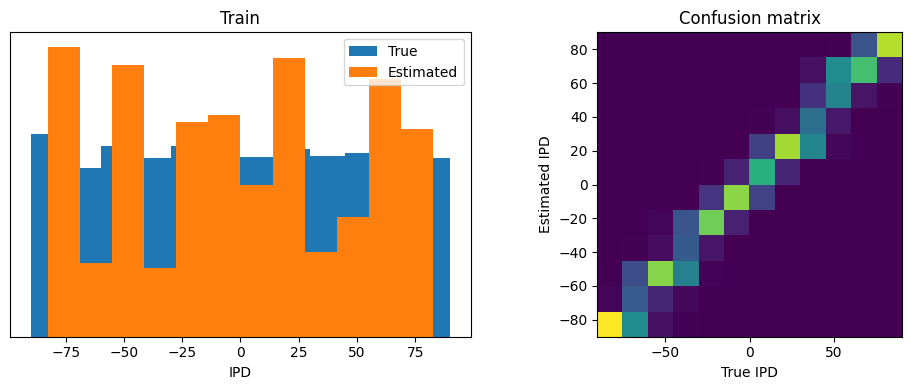
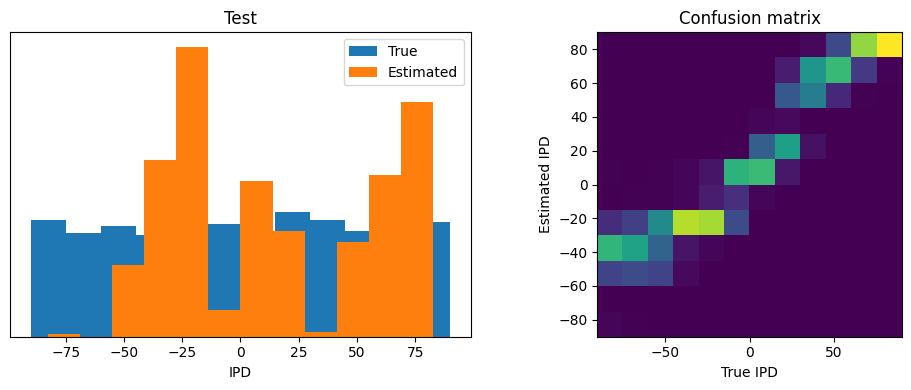
Results¶
Currently the model with the multiple contacts (here M=3), achieves marginally better training accuracy (65.6%) than the training accuracy (63.3%) of the no multiple contacts model (M=1), but its test accuracy is much worse (57.3% vs 31.1%).
However, it seems that the multiple contacts model overfits to the data. We should possibly add some regularization means, or check out the hyper-parameters we are using.