Imports¶
import numpy as np
import matplotlib.pyplot as plt
import matplotlib.colors
import matplotlib.cm
import torch
import torch.nn as nn
from tqdm.auto import tqdm as pbar
dtype = torch.float
if torch.cuda.is_available():
device = torch.device("cuda")
else:
device = torch.device("cpu")
from typing import Optional, Tuple
import math
Hyperparameters¶
# Constants
SECONDS = 1
MS = 1e-3
HZ = 1
DT = 1 * MS # large time step to make simulations run faster
ANF_PER_EAR = 100 # repeats of each ear with independent noise
DURATION = .1 * SECONDS # stimulus duration
DURATION_STEPS = int(np.round(DURATION / DT))
INPUT_SIZE = 2 * ANF_PER_EAR
# Training
LR = 0.001
N_EPOCHS = 150
batch_size = 64
n_training_batches = 64
n_testing_batches = 32
num_samples = batch_size*n_training_batches
# classes at 15 degree increments
NUM_CLASSES = 180 // 15
print(f'Number of classes = {NUM_CLASSES}')
# Network
NUM_HIDDEN = 30 # number of hidden units
TAU = 5 # membrane time constant
max_delay = 300//10
max_delay = max_delay if max_delay%2==1 else max_delay+1 # to make kernel_size an odd number
left_padding = max_delay-1
right_padding = (max_delay-1) // 2
Number of classes = 12
Functions¶
Stimulus¶
def input_signal(ipd, poisson, with_delays = False):
"""
Generate an input signal (spike array) from array of true IPDs
"""
envelope_power = 2 # higher values make sharper envelopes, easier
rate_max = 600 * HZ # maximum Poisson firing rate
stimulus_frequency = 20 * HZ
num_samples = len(ipd)
times = np.arange(DURATION_STEPS) * DT # array of times
phi = 2*np.pi*(stimulus_frequency * times + np.random.rand()) # array of phases corresponding to those times with random offset
# each point in the array will have a different phase based on which ear it is
theta = np.zeros((num_samples, DURATION_STEPS, 2*ANF_PER_EAR))
if with_delays:
# for each ear, we have anf_per_ear different phase delays from to pi/2 so
# that the differences between the two ears can cover the full range from -pi/2 to pi/2
phase_delays = np.linspace(0, np.pi/2, ANF_PER_EAR)
else:
phase_delays = np.zeros((ANF_PER_EAR))
# now we set up these theta to implement that. Some numpy vectorisation logic here which looks a little weird,
# but implements the idea in the text above.
theta[:, :, :ANF_PER_EAR] = phi[np.newaxis, :, np.newaxis]+phase_delays[np.newaxis, np.newaxis, :]
theta[:, :, ANF_PER_EAR:] = phi[np.newaxis, :, np.newaxis]+phase_delays[np.newaxis, np.newaxis, :]+ipd[:, np.newaxis, np.newaxis]
# now generate Poisson spikes at the given firing rate as in the previous notebook
if poisson is None:
poisson = np.random.rand(num_samples, DURATION_STEPS, 2*ANF_PER_EAR)
spikes = poisson<rate_max*DT*(0.5*(1+np.sin(theta)))**envelope_power
return spikes
def random_ipd_input_signal(num_samples, tensor=True):
"""
Generate the training data
Returns true IPDs from U(-pi/2, pi/2) and corresponding spike arrays
"""
ipd = np.random.rand(num_samples)*np.pi-np.pi/2 # uniformly random in (-pi/2, pi/2)
poisson = np.random.rand(num_samples, DURATION_STEPS, 2*ANF_PER_EAR)
if tensor:
ipd = torch.tensor(ipd, device=device, dtype=dtype)
return ipd, poisson
def spikes_from_fixed_idp_input_signal(ipd, poisson=None, tensor=True, with_delays=False):
spikes = input_signal(ipd, poisson, with_delays)
if tensor:
spikes = torch.tensor(spikes, device=device, dtype=dtype)
return spikes
def show_examples(shown=8):
ipd = np.linspace(-np.pi/2, np.pi/2, shown)
spikes = spikes_from_fixed_idp_input_signal(ipd, shown).cpu()
plt.figure(figsize=(10, 4), dpi=100)
for i in range(shown):
plt.subplot(2, shown // 2, i+1)
plt.imshow(spikes[i, :, :].T, aspect='auto', interpolation='nearest', cmap=plt.cm.gray_r)
plt.title(f'True IPD = {int(ipd[i]*180/np.pi)} deg')
if i>=4:
plt.xlabel('Time (steps)')
if i%4==0:
plt.ylabel('Input neuron index')
plt.tight_layout()
def data_generator(ipds, spikes):
perm = torch.randperm(spikes.shape[0])
spikes = spikes[perm, :, :]
ipds = ipds[perm]
n, _, _ = spikes.shape
n_batch = n//batch_size
for i in range(n_batch):
x_local = spikes[i*batch_size:(i+1)*batch_size, :, :]
y_local = ipds[i*batch_size:(i+1)*batch_size]
yield x_local, y_local
def discretise(ipds):
return ((ipds+np.pi/2) * NUM_CLASSES / np.pi).long() # assumes input is tensor
def continuise(ipd_indices): # convert indices back to IPD midpoints
return (ipd_indices+0.5) / NUM_CLASSES * np.pi - np.pi / 2
Without delays
# Plot a few just to show how it looks
ipds, poisson = random_ipd_input_signal(8, False)
spikes = spikes_from_fixed_idp_input_signal(ipds, poisson, True, False)
spikes = spikes.cpu()
plt.figure(figsize=(10, 4), dpi=100)
for i in range(8):
plt.subplot(2, 4, i+1)
plt.imshow(spikes[i, :, :].T, aspect='auto', interpolation='nearest', cmap=plt.cm.gray_r)
plt.title(f'True IPD = {int(ipds[i]*180/np.pi)} deg')
if i>=4:
plt.xlabel('Time (steps)')
if i%4==0:
plt.ylabel('Input neuron index')
plt.tight_layout()
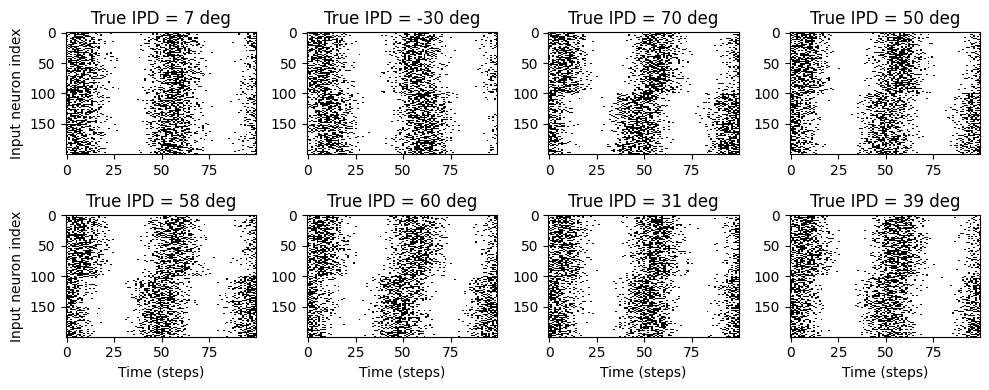
With delays
# Plot a few just to show how it looks
ipds, poisson = random_ipd_input_signal(8, False)
spikes = spikes_from_fixed_idp_input_signal(ipds, poisson, True, True)
spikes = spikes.cpu()
plt.figure(figsize=(10, 4), dpi=100)
for i in range(8):
plt.subplot(2, 4, i+1)
plt.imshow(spikes[i, :, :].T, aspect='auto', interpolation='nearest', cmap=plt.cm.gray_r)
plt.title(f'True IPD = {int(ipds[i]*180/np.pi)} deg')
if i>=4:
plt.xlabel('Time (steps)')
if i%4==0:
plt.ylabel('Input neuron index')
plt.tight_layout()
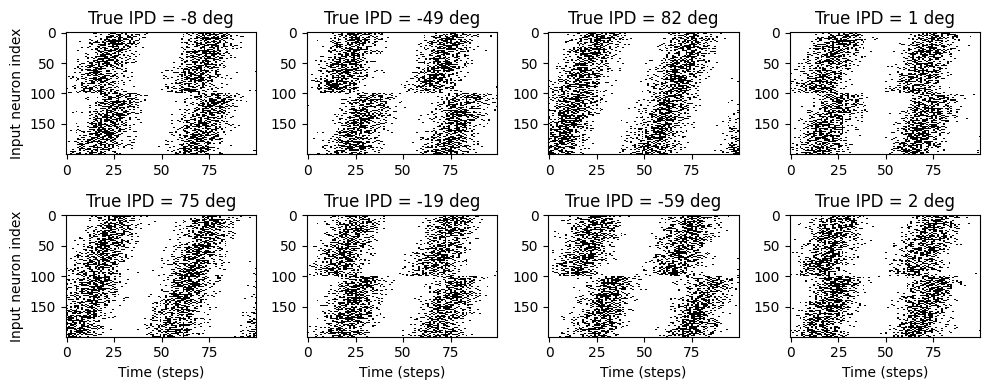
SNN¶
def sigmoid(x, beta):
return 1 / (1 + torch.exp(-beta*x))
def sigmoid_deriv(x, beta):
s = sigmoid(x, beta)
return beta * s * (1 - s)
class SurrGradSpike(torch.autograd.Function):
@staticmethod
def forward(ctx, inp):
ctx.save_for_backward(inp)
out = torch.zeros_like(inp)
out[inp > 0] = 1.0
return out
@staticmethod
def backward(ctx, grad_output):
inp, = ctx.saved_tensors
sigmoid_derivative = sigmoid_deriv(inp, beta=5)
grad = grad_output*sigmoid_derivative
return grad
spike_fn = SurrGradSpike.apply
def membrane_only(input_spikes, tau):
"""
:param input_spikes: has shape (batch_size, duration_steps, input_size)
:param weights: has shape (input_size, num_classes
:param tau:
:return:
"""
batch_size = input_spikes.shape[0]
assert len(input_spikes.shape) == 3
v = torch.zeros((batch_size, NUM_CLASSES), device=device, dtype=dtype)
v_rec = [v]
h = input_spikes
alpha = np.exp(-DT / tau)
for t in range(DURATION_STEPS - 1):
v = alpha*v + h[:, t, :]
v_rec.append(v)
v_rec = torch.stack(v_rec, dim=1) # (batch_size, duration_steps, num_classes)
return v_rec
def layer1(input_spikes, tau):
batch_size = input_spikes.shape[0]
# First layer: input to hidden
v = torch.zeros((batch_size, NUM_HIDDEN), device=device, dtype=dtype)
s = torch.zeros((batch_size, NUM_HIDDEN), device=device, dtype=dtype)
s_rec = [s]
h = input_spikes
alpha = np.exp(-DT / tau)
for t in range(DURATION_STEPS - 1):
new_v = (alpha*v + h[:, t, :])*(1-s) # multiply by 0 after a spike
s = spike_fn(v-1) # threshold of 1
v = new_v
s_rec.append(s)
s_rec = torch.stack(s_rec, dim=1)
return s_rec
def layer2(s_rec, tau):
"""Second layer: hidden to output"""
v_rec = membrane_only(s_rec, tau=tau)
return v_rec
def snn(input_spikes, w1, w2, tau=5*MS):
"""Run the simulation"""
x = input_spikes.permute(0,2,1)
x = nn.functional.pad(x, (left_padding, right_padding), 'constant', 0)
x = w1(x)
x = x.permute(0,2,1)
s_rec = layer1(x, tau)
x = s_rec.permute(0,2,1)
x = nn.functional.pad(x, (left_padding, right_padding), 'constant', 0)
x = w2(x)
x = x.permute(0,2,1)
v_rec = layer2(x, tau)
# Return recorded membrane potential of output
return v_rec
class _DclsNd(nn.modules.Module):
__constants__ = ['stride', 'padding', 'dilated_kernel_size', 'groups',
'padding_mode', 'output_padding', 'in_channels',
'out_channels', 'kernel_count', 'version']
__annotations__ = {'bias': Optional[torch.Tensor]}
def _conv_forward(self, input: torch.Tensor, weight: torch.Tensor, bias: Optional[torch.Tensor]) -> torch.Tensor:
...
_in_channels: int
out_channels: int
kernel_count: int
stride: Tuple[int, ...]
padding: Tuple[int, ...]
dilated_kernel_size: Tuple[int, ...]
transposed: bool
output_padding: Tuple[int, ...]
groups: int
padding_mode: str
weight: torch.Tensor
bias: Optional[torch.Tensor]
def __init__(self,
in_channels: int,
out_channels: int,
kernel_count: int,
stride: Tuple[int, ...],
padding: Tuple[int, ...],
dilated_kernel_size: Tuple[int, ...],
transposed: bool,
output_padding: Tuple[int, ...],
groups: int,
bias: bool,
padding_mode: str,
version: str) -> None:
super(_DclsNd, self).__init__()
if in_channels % groups != 0:
raise ValueError('in_channels must be divisible by groups')
if out_channels % groups != 0:
raise ValueError('out_channels must be divisible by groups')
valid_padding_modes = {'zeros', 'reflect', 'replicate', 'circular'}
if padding_mode not in valid_padding_modes:
raise ValueError("padding_mode must be one of {}, but got padding_mode='{}'".format(
valid_padding_modes, padding_mode))
self.in_channels = in_channels
self.out_channels = out_channels
self.kernel_count = kernel_count
self.stride = stride
self.padding = padding
self.dilated_kernel_size = dilated_kernel_size
self.transposed = transposed
self.output_padding = output_padding
self.groups = groups
self.padding_mode = padding_mode
self.version = version
# `_reversed_padding_repeated_twice` is the padding to be passed to
# `F.pad` if needed (e.g., for non-zero padding types that are
# implemented as two ops: padding + conv). `F.pad` accepts paddings in
# reverse order than the dimension.
self._reversed_padding_repeated_twice = nn.modules.utils._reverse_repeat_tuple(self.padding, 2)
if transposed:
self.weight = nn.parameter.Parameter(torch.Tensor(
in_channels, out_channels // groups, kernel_count))
self.P = nn.parameter.Parameter(torch.Tensor(len(dilated_kernel_size), in_channels,
out_channels // groups, kernel_count))
if version in ['gauss', 'max']:
self.SIG = nn.parameter.Parameter(torch.Tensor(len(dilated_kernel_size), in_channels,
out_channels // groups, kernel_count))
else:
self.register_parameter('SIG', None)
else:
self.weight = nn.parameter.Parameter(torch.Tensor(
out_channels, in_channels // groups, kernel_count))
self.P = nn.parameter.Parameter(torch.Tensor(len(dilated_kernel_size),
out_channels, in_channels // groups, kernel_count))
if version in ['gauss', 'max']:
self.SIG = nn.parameter.Parameter(torch.Tensor(len(dilated_kernel_size), out_channels,
in_channels // groups, kernel_count))
else:
self.register_parameter('SIG', None)
if bias:
self.bias = nn.parameter.Parameter(torch.empty(out_channels))
else:
self.register_parameter('bias', None)
self.reset_parameters()
def reset_parameters(self) -> None:
nn.init.kaiming_uniform_(self.weight, a=math.sqrt(5))
if self.bias is not None:
fan_in, _ = nn.init._calculate_fan_in_and_fan_out(self.weight)
bound = 1 / math.sqrt(fan_in)
nn.init.uniform_(self.bias, -bound, bound)
with torch.no_grad():
for i in range(len(self.dilated_kernel_size)):
lim = self.dilated_kernel_size[i] // 2
nn.init.normal_(self.P.select(0,i), 0, 0.5).clamp(-lim, lim)
if self.SIG is not None:
if self.version == 'gauss':
nn.init.constant_(self.SIG, 0.23)
else:
nn.init.constant_(self.SIG, 0.0)
def clamp_parameters(self) -> None:
for i in range(len(self.dilated_kernel_size)):
with torch.no_grad():
lim = self.dilated_kernel_size[i] // 2
self.P.select(0,i).clamp_(-lim, lim)
def extra_repr(self):
s = ('{in_channels}, {out_channels}, kernel_count={kernel_count} (previous kernel_size)'
', stride={stride}, version={version}')
if self.padding != (0,) * len(self.padding):
s += ', padding={padding}'
if self.dilated_kernel_size != (1,) * len(self.dilated_kernel_size):
s += ', dilated_kernel_size={dilated_kernel_size} (learnable)'
if self.output_padding != (0,) * len(self.output_padding):
s += ', output_padding={output_padding}'
if self.groups != 1:
s += ', groups={groups}'
if self.bias is None:
s += ', bias=False'
if self.padding_mode != 'zeros':
s += ', padding_mode={padding_mode}'
return s.format(**self.__dict__)
def __setstate__(self, state):
super(_DclsNd, self).__setstate__(state)
if not hasattr(self, 'padding_mode'):
self.padding_mode = 'zeros'
class ConstructKernel1d(nn.modules.Module):
def __init__(self, out_channels, in_channels, groups, kernel_count, dilated_kernel_size, version):
super().__init__()
self.version = version
self.out_channels = out_channels
self.in_channels = in_channels
self.groups = groups
self.dilated_kernel_size = dilated_kernel_size
self.kernel_count = kernel_count
self.IDX = None
self.lim = None
def __init_tmp_variables__(self, device):
if self.IDX is None or self.lim is None:
I = nn.parameter.Parameter(torch.arange(0, self.dilated_kernel_size[0]), requires_grad=False).to(device)
IDX = I.unsqueeze(0)
IDX = IDX.expand(self.out_channels, self.in_channels//self.groups, self.kernel_count, -1, -1).permute(4,3,0,1,2)
self.IDX = IDX
lim = torch.tensor(self.dilated_kernel_size).to(device)
self.lim = lim.expand(self.out_channels, self.in_channels//self.groups, self.kernel_count, -1).permute(3,0,1,2)
else:
pass
def forward_vmax(self, W, P, SIG):
P = P + self.lim // 2
SIG = SIG.abs() + 1.0
X = (self.IDX - P)
X = ((SIG - X.abs()).relu()).prod(1)
X = X / (X.sum(0) + 1e-7) # normalization
K = (X * W).sum(-1)
K = K.permute(1,2,0)
return K
def forward_vgauss(self, W, P, SIG):
P = P + self.lim // 2
SIG = SIG.abs() + 0.27
X = ((self.IDX - P) / SIG).norm(2, dim=1)
X = (-0.5 * X**2).exp()
X = X / (X.sum(0) + 1e-7) # normalization
K = (X * W).sum(-1)
K = K.permute(1,2,0)
return K
def forward(self, W, P, SIG):
self.__init_tmp_variables__(W.device)
if self.version == 'max':
return self.forward_vmax(W, torch.clamp(torch.round(P), -(self.dilated_kernel_size[0] // 2), (self.dilated_kernel_size[0]// 2)), torch.zeros_like(SIG))
elif self.version == 'gauss':
return self.forward_vgauss(W, P, SIG)
else:
raise
def extra_repr(self):
s = ('{in_channels}, {out_channels}, kernel_count={kernel_count}, version={version}')
if self.dilated_kernel_size != (1,) * len(self.dilated_kernel_size):
s += ', dilated_kernel_size={dilated_kernel_size}'
if self.groups != 1:
s += ', groups={groups}'
return s.format(**self.__dict__)
class Dcls1d(_DclsNd):
__doc__ = r"""
Shape:
- Input: :math:`(N, C_{in}, L_{in})` or :math:`(C_{in}, L_{in})`
- Output: :math:`(N, C_{out}, L_{out})` or :math:`(C_{out}, L_{out})`, where
.. math::
L_{out} = \left\lfloor\frac{L_{in} + 2 \times \text{padding} - \text{dilation}
\times (\text{kernel\_size} - 1) - 1}{\text{stride}} + 1\right\rfloor
Attributes:
weight (Tensor): the learnable weights of the module of shape
:math:`(\text{out\_channels},
\frac{\text{in\_channels}}{\text{groups}}, \text{kernel\_size})`.
The values of these weights are sampled from
:math:`\mathcal{U}(-\sqrt{k}, \sqrt{k})` where
:math:`k = \frac{groups}{C_\text{in} * \text{kernel\_size}}`
bias (Tensor): the learnable bias of the module of shape
(out_channels). If :attr:`bias` is ``True``, then the values of these weights are
sampled from :math:`\mathcal{U}(-\sqrt{k}, \sqrt{k})` where
:math:`k = \frac{groups}{C_\text{in} * \text{kernel\_size}}`
Examples::
>>> m = nn.Conv1d(16, 33, 3, stride=2)
>>> input = torch.randn(20, 16, 50)
>>> output = m(input)
.. _cross-correlation:
https://en.wikipedia.org/wiki/Cross-correlation
.. _link:
https://github.com/vdumoulin/conv_arithmetic/blob/master/README.md
"""
def __init__(
self,
in_channels: int,
out_channels: int,
kernel_count: int,
stride: nn.common_types._size_1_t = 1,
padding: nn.common_types._size_1_t = 0,
dilated_kernel_size: nn.common_types._size_1_t = 1,
groups: int = 1,
bias: bool = True,
padding_mode: str = 'zeros', # TODO: refine this type
version: str = 'v1'
):
stride_ = nn.modules.utils._single(stride)
padding_ = nn.modules.utils._single(padding)
dilated_kernel_size_ = nn.modules.utils._single(dilated_kernel_size)
super(Dcls1d, self).__init__(
in_channels, out_channels, kernel_count, stride_, padding_, dilated_kernel_size_,
False, nn.modules.utils._single(0), groups, bias, padding_mode, version)
self.DCK = ConstructKernel1d(self.out_channels,
self.in_channels,
self.groups,
self.kernel_count,
self.dilated_kernel_size,
self.version)
def extra_repr(self):
s = super(Dcls1d, self).extra_repr()
return s.format(**self.__dict__)
def _conv_forward(self, input: torch.Tensor, weight: torch.Tensor, bias: Optional[torch.Tensor], P: torch.Tensor, SIG: Optional[torch.Tensor]):
if self.padding_mode != 'zeros':
return nn.functional.conv1d(nn.functional.pad(input, self._reversed_padding_repeated_twice, mode=self.padding_mode),
self.DCK(weight, P, SIG), bias,
self.stride, nn.modules.utils._single(0), nn.modules.utils._single(1), self.groups)
return nn.functional.conv1d(input, self.DCK(weight, P, SIG), bias,
self.stride, self.padding, nn.modules.utils._single(1), self.groups)
def forward(self, input: torch.Tensor) -> torch.Tensor:
return self._conv_forward(input, self.weight, self.bias, self.P, self.SIG)
def init_weight_matrices():
"""Weights initialisation"""
w1 = Dcls1d(INPUT_SIZE, NUM_HIDDEN, kernel_count=1, groups = 1, dilated_kernel_size = max_delay, bias=False, version='gauss')
w2 = Dcls1d(NUM_HIDDEN, NUM_CLASSES, kernel_count=1, groups = 1, dilated_kernel_size = max_delay, bias=False, version='gauss')
nn.init.kaiming_uniform_(w1.weight, nonlinearity='relu')
nn.init.kaiming_uniform_(w2.weight, nonlinearity='relu')
torch.nn.init.constant_(w1.SIG, max_delay // 2 )
w1.SIG.requires_grad = False
torch.nn.init.constant_(w2.SIG, max_delay // 2 )
w2.SIG.requires_grad = False
return w1, w2
Training¶
def train(w1, w2, ipds, poisson, ipds_validation, poisson_validation, lr=0.001, n_epochs=150, tau=5*MS):
"""
:param lr: learning rate
:return:
"""
# Optimiser and loss function
positions = []
weights = []
positions.append(w1.P)
weights.append(w1.weight)
positions.append(w2.P)
weights.append(w2.weight)
optimizers = []
optimizers.append(torch.optim.Adam([{'params':weights, 'lr':lr}]))
optimizers.append(torch.optim.Adam(positions, lr = lr * 100))
schedulers = []
schedulers.append(torch.optim.lr_scheduler.OneCycleLR(optimizers[0], max_lr=5*lr, total_steps=n_epochs))
schedulers.append(torch.optim.lr_scheduler.CosineAnnealingLR(optimizers[1], T_max=n_epochs))
log_softmax_fn = nn.LogSoftmax(dim=1)
loss_fn = nn.NLLLoss()
loss_hist = []
val_loss_hist = []
best_loss = 1e10
val_loss_best_loss = 1e10
for e in pbar(range(n_epochs)):
local_loss = []
spikes = spikes_from_fixed_idp_input_signal(ipds, poisson)
for x_local, y_local in data_generator(discretise(torch.tensor(ipds, device=device, dtype=dtype)), spikes):
# Run network
output = snn(x_local, w1, w2, tau=tau)
# Compute cross entropy loss
m = torch.sum(output, 1)*0.01 # Sum time dimension
reg = 0
loss = loss_fn(log_softmax_fn(m), y_local) + reg
local_loss.append(loss.item())
# Update gradients
for opt in optimizers: opt.zero_grad()
loss.backward()
for opt in optimizers: opt.step()
w1.clamp_parameters()
w2.clamp_parameters()
loss_hist.append(np.mean(local_loss))
for scheduler in schedulers: scheduler.step()
alpha = 0
sig = w2.SIG[0,0,0,0].detach().cpu().item()
if e < ((1*n_epochs)//4) and sig > 0.23:
alpha = (0.23/(max_delay // 2 ))**(1/(((1*n_epochs)//4)))
w1.SIG *= alpha
w2.SIG *= alpha
val_local_loss = []
with torch.no_grad():
w1.version = 'max'
w1.DCK.version = 'max'
w2.version = 'max'
w2.DCK.version = 'max'
w1.clamp_parameters()
w2.clamp_parameters()
spikes_validation = spikes_from_fixed_idp_input_signal(ipds_validation, poisson_validation)
for x_local, y_local in data_generator(discretise(torch.tensor(ipds_validation, device=device, dtype=dtype)), spikes_validation):
# Run network
output = snn(x_local, w1, w2, tau=tau)
# Compute cross entropy loss
m = torch.sum(output, 1)*0.01 # Sum time dimension
val_loss = loss_fn(log_softmax_fn(m), y_local)
val_local_loss.append(val_loss.item())
w1.clamp_parameters()
w2.clamp_parameters()
w1.version = 'gauss'
w1.DCK.version = 'gauss'
w2.version = 'gauss'
w2.DCK.version = 'gauss'
val_loss_hist.append(np.mean(val_local_loss))
if np.mean(val_local_loss) < val_loss_best_loss:
val_loss_best_loss = np.mean(val_local_loss)
best_weights = w1, w2
#Early Stopping :
if torch.tensor(val_loss_hist[-10:]).argmin() == 0 and e>10:
print('Early Stop !')
return best_weights
# Plot the loss function over time
plt.plot(loss_hist)
plt.xlabel('Epoch')
plt.ylabel('Loss')
plt.tight_layout()
plt.plot(val_loss_hist)
plt.xlabel('Epoch')
plt.ylabel('Loss')
plt.tight_layout()
return w1, w2
Testing¶
def test_accuracy(ipds, poisson, run):
accs = []
ipd_true = []
ipd_est = []
confusion = np.zeros((NUM_CLASSES, NUM_CLASSES))
spikes = spikes_from_fixed_idp_input_signal(ipds, poisson)
for x_local, y_local in data_generator((torch.tensor(ipds, device=device, dtype=dtype)), spikes):
y_local_orig = y_local
y_local = discretise(y_local)
output = run(x_local)
m = torch.sum(output, 1) # Sum time dimension
_, am = torch.max(m, 1) # argmax over output units
tmp = np.mean((y_local == am).detach().cpu().numpy()) # compare to labels
for i, j in zip(y_local.detach().cpu().numpy(), am.detach().cpu().numpy()):
confusion[j, i] += 1
ipd_true.append(y_local_orig.cpu().data.numpy())
ipd_est.append(continuise(am.detach().cpu().numpy()))
accs.append(tmp)
ipd_true = np.hstack(ipd_true)
ipd_est = np.hstack(ipd_est)
return ipd_true, ipd_est, confusion, accs
def report_accuracy(ipd_true, ipd_est, confusion, accs, label):
abs_errors_deg = abs(ipd_true-ipd_est)*180/np.pi
print()
print(f"{label} classifier accuracy: {100*np.mean(accs):.1f}%")
print(f"{label} absolute error: {np.mean(abs_errors_deg):.1f} deg")
plt.figure(figsize=(10, 4), dpi=100)
plt.subplot(121)
plt.hist(ipd_true * 180 / np.pi, bins=NUM_CLASSES, label='True')
plt.hist(ipd_est * 180 / np.pi, bins=NUM_CLASSES, label='Estimated')
plt.xlabel("IPD")
plt.yticks([])
plt.legend(loc='best')
plt.title(label)
plt.subplot(122)
confusion /= np.sum(confusion, axis=0)[np.newaxis, :]
plt.imshow(confusion, interpolation='nearest', aspect='equal', origin='lower', extent=(-90, 90, -90, 90))
plt.xlabel('True IPD')
plt.ylabel('Estimated IPD')
plt.title('Confusion matrix')
plt.tight_layout()
def analyse_accuracy(ipds, poisson, run, label):
ipd_true, ipd_est, confusion, accs = test_accuracy(ipds, poisson, run)
report_accuracy(ipd_true, ipd_est, confusion, accs, label)
return 100*np.mean(accs)
Train Network¶
# Generate the training data
w1, w2 = init_weight_matrices()
ipds_training, poisson_training = random_ipd_input_signal(num_samples, False)
ipds_validation, poisson_validation = random_ipd_input_signal(num_samples, False)
# Train network
w1_trained, w2_trained = train(w1=w1, w2=w2, ipds=ipds_training, poisson=poisson_training, poisson_validation=poisson_validation, ipds_validation=ipds_validation, lr=LR, n_epochs=N_EPOCHS, tau=TAU*MS)
Loading...
with torch.no_grad():
w1_trained.version = 'max'
w1_trained.DCK.version = 'max'
w2_trained.version = 'max'
w2_trained.DCK.version = 'max'
# Analyse
print(f"Chance accuracy level: {100 * 1 / NUM_CLASSES:.1f}%")
run_func = lambda x: snn(x, w1_trained, w2_trained)
analyse_accuracy(ipds_training, poisson_training, run_func, 'Train')
ipds_test, poisson_test = random_ipd_input_signal(batch_size*n_testing_batches, False)
analyse_accuracy(ipds_test, poisson_test, run_func, 'Test')
Chance accuracy level: 8.3%
Train classifier accuracy: 89.9%
Train absolute error: 4.1 deg
Test classifier accuracy: 88.2%
Test absolute error: 4.2 deg
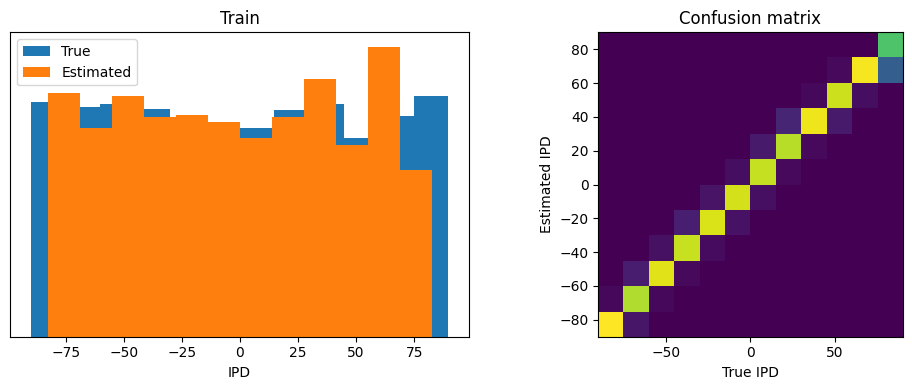
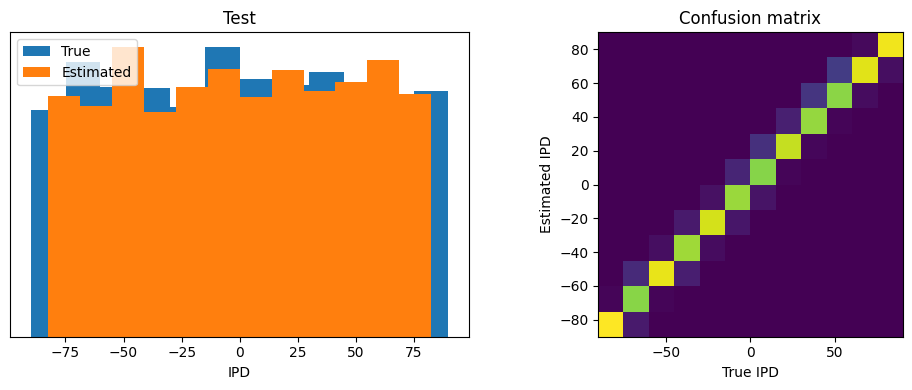
w1_delay = w1_trained.P.squeeze().detach().round_()
print("Minimum: ", torch.min(w1_delay), "Maximum: ", torch.max(w1_delay), "Mean: ", torch.mean(w1_delay), "STD: ", torch.std(w1_delay))
Minimum: tensor(-15.) Maximum: tensor(15.) Mean: tensor(6.5355) STD: tensor(7.2640)
plt.imshow(w1_delay.numpy())
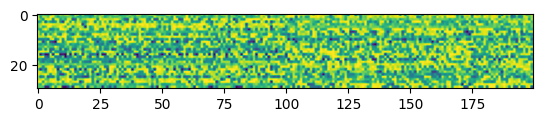
w2_delay = w2_trained.P.squeeze().detach().round_()
print("Minimum: ", torch.min(w2_delay), "Maximum: ", torch.max(w2_delay), "Mean: ", torch.mean(w2_delay), "STD: ", torch.std(w2_delay))
Minimum: tensor(-15.) Maximum: tensor(15.) Mean: tensor(4.8556) STD: tensor(10.8649)
plt.imshow(w2_delay.numpy())
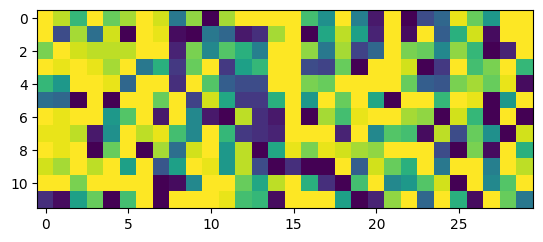
Now go from 30ms max delay to 25ms
max_delay = 250//10
max_delay = max_delay if max_delay%2==1 else max_delay+1 # to make kernel_size an odd number
left_padding = max_delay-1
right_padding = (max_delay-1) // 2
# Generate the training data
w1, w2 = init_weight_matrices()
# Train network
w1_trained, w2_trained = train(w1=w1, w2=w2, ipds=ipds_training, poisson=poisson_training, poisson_validation=poisson_validation, ipds_validation=ipds_validation, lr=LR, n_epochs=N_EPOCHS, tau=TAU*MS)
Loading...
with torch.no_grad():
w1_trained.version = 'max'
w1_trained.DCK.version = 'max'
w2_trained.version = 'max'
w2_trained.DCK.version = 'max'
# Analyse
print(f"Chance accuracy level: {100 * 1 / NUM_CLASSES:.1f}%")
run_func = lambda x: snn(x, w1_trained, w2_trained)
analyse_accuracy(ipds_training, poisson_training, run_func, 'Train')
analyse_accuracy(ipds_test, poisson_test, run_func, 'Test')
Chance accuracy level: 8.3%
Train classifier accuracy: 80.3%
Train absolute error: 4.8 deg
Test classifier accuracy: 81.8%
Test absolute error: 4.6 deg
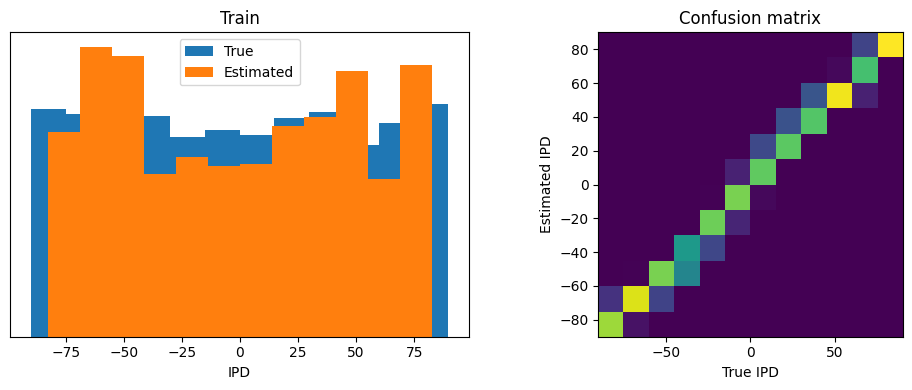
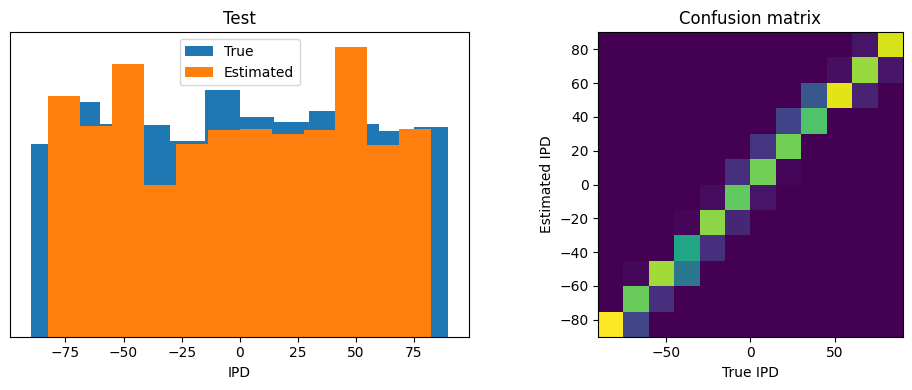
w1_delay = w1_trained.P.squeeze().detach().round_()
print("Minimum: ", torch.min(w1_delay), "Maximum: ", torch.max(w1_delay), "Mean: ", torch.mean(w1_delay), "STD: ", torch.std(w1_delay))
Minimum: tensor(-12.) Maximum: tensor(12.) Mean: tensor(3.8682) STD: tensor(6.5771)
plt.imshow(w1_delay.numpy())
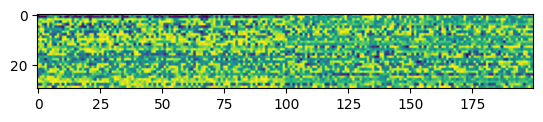
w2_delay = w2_trained.P.squeeze().detach().round_()
print("Minimum: ", torch.min(w2_delay), "Maximum: ", torch.max(w2_delay), "Mean: ", torch.mean(w2_delay), "STD: ", torch.std(w2_delay))
Minimum: tensor(-12.) Maximum: tensor(12.) Mean: tensor(4.1833) STD: tensor(8.6819)
plt.imshow(w2_delay.numpy())
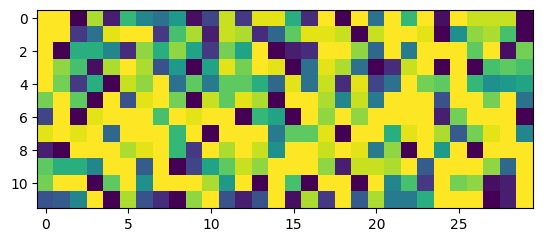
Train accuracy is slightly lower than test..Seems like 25 is too small , let’s see 35
max_delay = 350//10
max_delay = max_delay if max_delay%2==1 else max_delay+1 # to make kernel_size an odd number
left_padding = max_delay-1
right_padding = (max_delay-1) // 2
# Generate the training data
w1, w2 = init_weight_matrices()
# Train network
w1_trained, w2_trained = train(w1=w1, w2=w2, ipds=ipds_training, poisson=poisson_training, poisson_validation=poisson_validation, ipds_validation=ipds_validation, lr=LR, n_epochs=N_EPOCHS, tau=TAU*MS)
Loading...
with torch.no_grad():
w1_trained.version = 'max'
w1_trained.DCK.version = 'max'
w2_trained.version = 'max'
w2_trained.DCK.version = 'max'
# Analyse
print(f"Chance accuracy level: {100 * 1 / NUM_CLASSES:.1f}%")
run_func = lambda x: snn(x, w1_trained, w2_trained)
analyse_accuracy(ipds_training, poisson_training, run_func, 'Train')
analyse_accuracy(ipds_test, poisson_test, run_func, 'Test')
Chance accuracy level: 8.3%
Train classifier accuracy: 85.1%
Train absolute error: 4.4 deg
Test classifier accuracy: 84.4%
Test absolute error: 4.4 deg
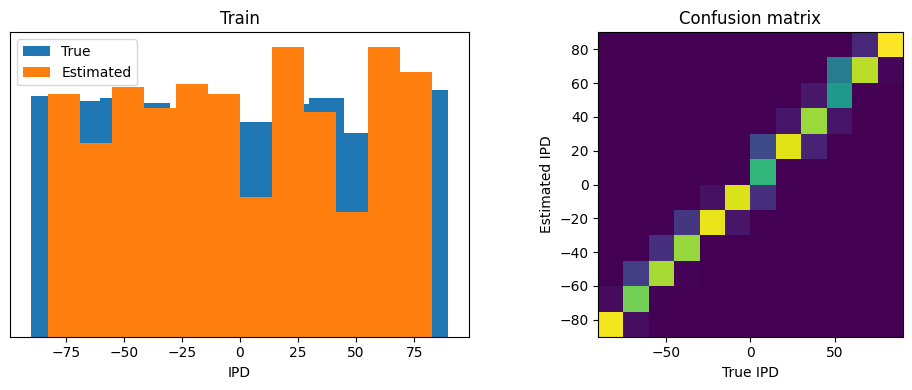
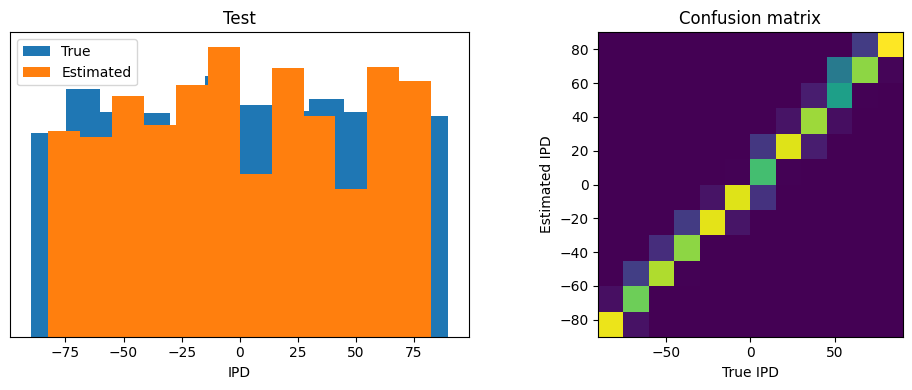
w1_delay = w1_trained.P.squeeze().detach().round_()
print("Minimum: ", torch.min(w1_delay), "Maximum: ", torch.max(w1_delay), "Mean: ", torch.mean(w1_delay), "STD: ", torch.std(w1_delay))
Minimum: tensor(-17.) Maximum: tensor(17.) Mean: tensor(5.5645) STD: tensor(9.3541)
plt.imshow(w1_delay.numpy())
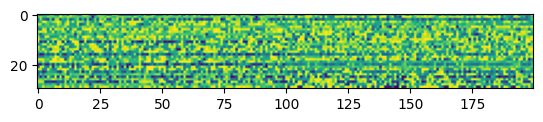
w2_delay = w2_trained.P.squeeze().detach().round_()
print("Minimum: ", torch.min(w2_delay), "Maximum: ", torch.max(w2_delay), "Mean: ", torch.mean(w2_delay), "STD: ", torch.std(w2_delay))
Minimum: tensor(-17.) Maximum: tensor(17.) Mean: tensor(6.9472) STD: tensor(12.1914)
plt.imshow(w2_delay.numpy())
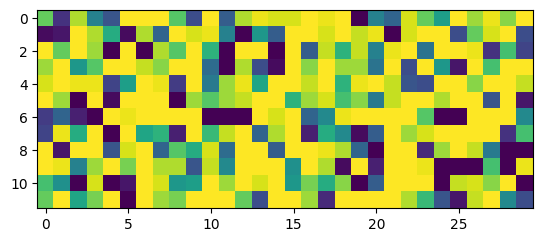
35 seems like too much